Opening up AI access to unlock decision making [Q&A]

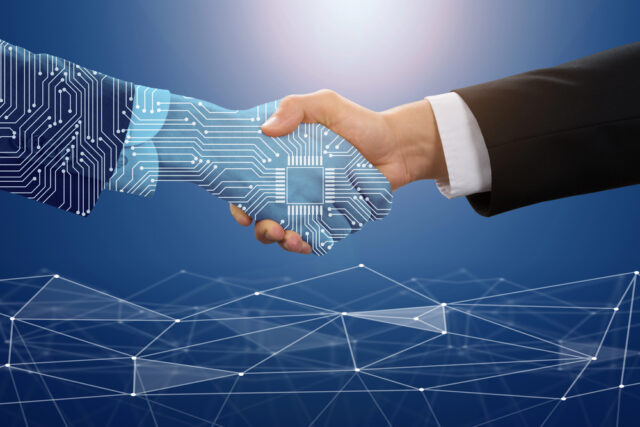
More and more businesses are turning to generative AI in the hope of gaining greater value from their data. But there are issues around governance, access to data and lack of appropriate skills that mean projects can either stall or not deliver as expected.
We spoke to Srujan Akula, CEO of The Modern Data Company, about why he believes that giving 'power to the people' and democratizing AI by putting it in the hands of non-technical users can deliver real business value.
BN: Why are organizations failing to obtain the best value from AI?
SA: The biggest roadblock to AI success isn't actually about AI at all, it's about data quality and management. While companies race to integrate sophisticated AI models, they're hitting the classic 'garbage in, garbage out' problem on an enterprise scale. Organizations face three critical challenges that prevent their AI investments from delivering real value:
First, data quality and context. AI requires clean, trusted data, but most enterprise data is raw and fragmented across multiple sources. Without proper contextualization and cleansing, it's nearly impossible to effectively apply AI.
Second, data discovery and accessibility. Data engineers spend most of their time just trying to locate and format the right data rather than creating value with it. This leads to the infamous '80/20 rule' where 80 percent of time is spent on data engineering tasks rather than actual modeling and analysis.
Finally, governance complexity. Organizations struggle to properly protect their data while making it useful. Without a comprehensive knowledge graph and proper governance framework, it's difficult to determine what data an AI model should have access to and how it should be protected.
BN: How can giving wider access to AI tools deliver benefits?
SA: AI tools can create a powerful multiplier effect across the organization. At The Modern Data Company, we're seeing this play out in several ways:
We use ML and AI to quickly assess data quality, understand data profiles, and identify classification patterns. This helps automate traditionally manual tasks like identifying personally identifiable information (PII) in free text.
More importantly, AI can actually improve your data quality over time. It can identify gaps, inconsistencies, and potential areas for improvement. We've developed AI-driven personas that can automate routine data engineering tasks, allowing teams to focus on value creation.
With generative AI, we're taking this further by implementing co-pilot capabilities that can auto-discover metadata and provide deeper insights into data relationships and usage patterns.
BN: Isn't there a problem with enterprises having siloed data that's hard to access?
SA: Yes, there is. This remains one of the most significant challenges in enterprise data management. Traditional approaches like maintaining data catalogs in spreadsheets are no longer sufficient. The solution requires a three-pronged approach:
- Automated data catalog creation with semantic search capabilities
- Intent-based discovery systems that understand what users are trying to accomplish
- Granular access control that maintains security while enabling data discovery
With Modern's DataOS, we've made this a core design principle. Our platform enables users to search for any data they need while maintaining strict governance through policy-based access controls. When schemas change or new data is added, it automatically becomes part of the discoverable asset base, all while maintaining appropriate access controls at the team level.
BN: What are the risks of doing this in terms of data governance and how can they be addressed?
SA: The fact that data exists shouldn't be a secret–what matters is controlling who can access it and how. The key insight here is that data visibility and data access are two different things. In fact, making data more discoverable while maintaining strict access controls often results in better security than keeping data hidden in silos.
We focus on metadata governance rather than complete data lockdown. This allows organizations to understand what data exists and who can access it, making data both more accessible and more secure. It might sound counterintuitive, but this approach actually strengthens both data utility and protection by providing clear visibility into data assets while maintaining rigorous access controls.
BN: Could opening up AI eliminate the need for data engineering or will the role evolve?
SA: AI won't eliminate data engineering any more than it will eliminate software engineering. Instead, it will transform the role to focus on higher-value activities. We're already seeing this evolution:
Data engineers are gaining significant productivity improvements through AI assistance. Instead of repeatedly building basic pipelines or writing boilerplate connection code, they can leverage AI assistants to handle routine tasks while focusing on strategic work.
The future data engineer will be less focused on maintaining infrastructure and more on driving business transformation through data innovation. As AI becomes central to business strategy, data engineers will evolve from pipeline builders to strategic partners in business transformation, focusing on turning data into actual business value rather than just managing it.
Image credit: AndreyPopov/depositphotos.com