How agentic AI takes GenAI to the next level [Q&A]

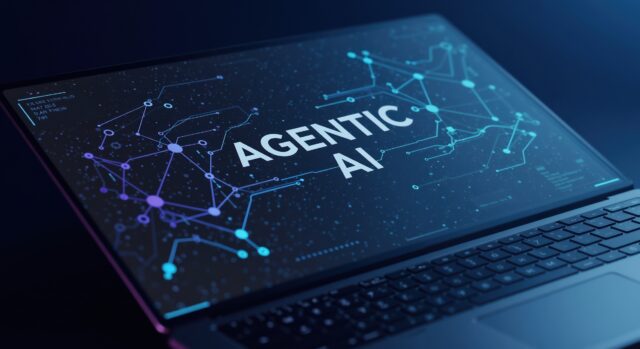
Agentic AI has been in the news quite a bit of late, but how should enterprises expect it to impact their organizations?
We spoke to Mike Finley, CTO of AnswerRocket, to discuss Agentic AI's benefits, use cases and more.
BN: What sort of use cases will agentic AI support?
MF: This is the assembly line moment for knowledge work. Just like during the Industrial Revolution, we have to decompose the work that people do into repeatable subtasks so they can be done more efficiently with automation. Before agentic AI, we assumed this decomposition would be impossible. But now, it's obvious.
Suppose we're processing customer complaints. We can have AI-driven stages to understand the problem, research the specifics of the case, trend issues with the product in question, compose an appropriate reply -- all in the corporate tone and brand voice. That was 100 percent human work two years ago. Now we'd be foolish to trust most humans to get it right every time.
Can it replace a surgeon, or the captain of a ship, or your kid's kindergarten teacher? Not for a long while. The barriers to interacting with the real world are thorny. We have not yet had the 'GPT moment' for robotics, but it will come. Unlike GPT, however, robotics won't scale cheaply into the millions of users, and they can’t wait seconds for a brain in the cloud to react.
BN: What benefits does it enable, and what types of organizations stand to benefit?
MF: There are tasks like help desks where people have lousy jobs doing the same thing over and over, ironically assisting customers that think the service is lousy and slow too, at a cost to enterprises that hurts profitability without adding competitive value. Everybody loses but we tolerate it because we’ve become accustomed to it. If we could serve all the customers better, faster, and cheaper, in an accountable framework that improves over time, everybody will win.
Agentic AI does just that. Any enterprise workload that does not physically touch the real world can likely be automated now. Anything we would call 'back office' is a great candidate. For example, most of the work involving ERP’s. As models gain more tools and agents get additional guardrails, we will entrust them with more and more tasks.
Enterprises should look for work that has a large number of people doing the same thing over and over. When an agent is implemented, the result will be a faster, better and more accountable gear in the enterprise machine. An easy way to identify these areas is to find cost centers that have wage-dominated financials. Any personnel cost that scales linearly with the top line of your business should be a candidate to be streamlined via AI agents.
BN: What are the data requirements and other pre-requisites to building AI agents?
MF: If you could hire an average college sophomore and give them training to do an office job, you already have the data you need for an AI agent. Large models can consume any content you would give a human, including training videos, web sites, documents and learning to use desktop IT tools. Don't get hung up in building and cleaning new data sets. Get the AI online then start making it better. It can already be more consistent, more available, cheaper and more coachable than people. Instead, get the people focused on the toughest use cases, the ones that touch the real world. Like planning for future initiatives, plugging in to real world trends, enforcing very human policies that require judgment and accountability.
To put agents in place, all you need is a framework to connect to your enterprise systems. If you have a tech stack like Microsoft or Salesforce, integrate there. If not, you can use AI to help you decide how. This space is moving fast so start soon and iterate. That sounds counterintuitive in areas like IT where we've been trained to go slow, plan a roadmap and test extensively. We do have to start small, but not slow. We need a roadmap, but it has to be along the lines of 'invest X to get Y' instead of 'upgrade to version N' like we have in the past.
BN: How will agentic AI impact the developer experience?
MF: AI is a boost for software development of any kind. The developer experience has forever changed with the advent of programs that can create, troubleshoot and update other software. All the pre-industrial coding concepts like 'low-code' are a thing of the past because AI can do that work. If you needed a code block to handle complex conditions, just replace it with a prompt. Prompts are the new source code. Natural Language is programming.
The real question is how do behavioral examples change the developer experience. The heartbeat of an AI agent comes from the examples that teach it how to do its job. An AI engineer's role is to divide the work of the AI such that inexpensive, fast models can get the job done in easy steps. Whether learning the firm but conciliatory tone of a collections agent, or the subtleties of how to address corrupted data, or the exquisite patterns of how to price commodities, it all comes down to the examples. After all, the pre-training of the model is just an average of, well, everything. So if you want your model to differentiate your business from others, your training examples are what will bring it above the rest.
BN: How can organizations test AI agents for accuracy?
MF: First, we can require AI Agents to provide proof points. That means any facts they use or quote have to be documented with sources that can be verified without AI. Any decisions made have to be documented with logical steps describing what inputs were used. Just like with human workers, this requirement seems to make the AI do a better job.
A second stage is the agentic AI concept of 'verifiers.' These are AI supervisor agents whose job is to watch the work of others and ensure that they fall in line. We're not just looking for accuracy, we’re looking for subtle things like tone and other cues. If we want these agents to do human work, we have to watch them like we would human workers.
Image Credit: Twoapril Studio/Dreamstime.com