Navigating data privacy and security challenges in AI [Q&A]

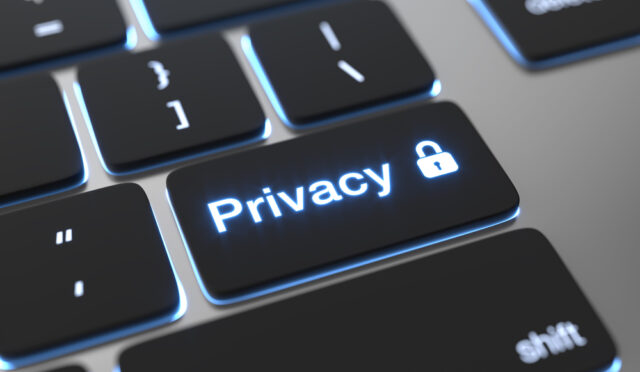
As artificial intelligence (AI) continues to reshape industries, data privacy and security concerns are escalating. The rapid growth of AI applications presents new challenges for companies in safeguarding sensitive information.
Emerging advanced AI models like Deepseek, developed outside the US, underscore the risks of handling critical data. We spoke to Amar Kanagaraj, CEO of Protecto -- a data guardrail company focused on AI security and privacy -- to get his insights on the most pressing AI data protection challenges.
BN: How does AI impact data security and privacy? What are the key risks?
AK: AI fundamentally changes how applications are developed and used. Interactions are shifting from fixed, rule-based systems to dynamic, conversational AI that continuously learns from new data. This evolving nature increases the surface area where data can be exposed, creating vulnerabilities that traditional security measures like role-based access controls and encryption may not fully address. Continuous learning cycles can inadvertently expose sensitive data, and legacy security measures such as encryption and role-based access often fall short in dynamic AI environments.
Additionally, the integration of AI into multiple workflows expands potential entry points for data breaches, increasing the overall risk landscape. Importantly, internal risks such as unauthorized access by employees or unintended data exposure through AI models are becoming more prevalent, emphasizing the need for comprehensive internal data security protocols.
BN: What data security measures should organizations implement when using AI?
AK: Organizations must adopt a multi-layered approach to AI security to mitigate these risks, with a strong focus on data security. Protecting sensitive information in AI use cases requires advanced data masking and anonymization techniques that ensure data utility while reducing exposure.
Additionally, implementing AI-specific guardrails is vital, including strategies to counter model inversion attacks, data poisoning, and membership inference attacks. Strong access controls should be enforced not only at the system level but also at the data and model levels to prevent unauthorized internal access. Organizations should establish robust data governance frameworks, implement strict monitoring of user activities, and maintain comprehensive audits to identify and mitigate potential internal threats alongside external ones.
BN: Does using on-premise or private large language models (LLMs) eliminate all risks?
AK: Not entirely. While on-premise models reduce certain risks associated with third-party access, they do not eliminate risks related to data distribution across development, testing, and actual usage phases. AI agents often interact with a wide variety of data sources within an organization, creating potential vulnerabilities regardless of where the models are deployed. The dynamic nature of AI operations means that data can still be exposed through various touchpoints, including internal channels where data might be accessed improperly. This emphasizes the need for holistic data protection strategies that address both external and internal threats.
BN: Can AI apps pose privacy risks even if the data is secured?
AK: Yes, AI apps can pose privacy risks even when the data is secured. The level of risk depends on various factors, including the hosting environment and the robustness of data governance policies. Apps using LLMs hosted on public clouds may face different risks compared to those deployed on-premise. Even without direct access to training data, attackers can sometimes extract sensitive information through sophisticated inference attacks.
Beyond external threats, internal risks also pose significant challenges. Employees with legitimate access to AI agents might misuse them to uncover sensitive details, either intentionally or unintentionally. Additionally, AI apps themselves can inadvertently expose data from one user to another, especially in multi-tenant environments.
These risks highlight the importance of strong policy enforcement, comprehensive security measures, rigorous access controls, and continuous monitoring to safeguard AI apps against both external and internal privacy breaches.
BN: What future trends should we watch in AI data privacy and security?
AK: The future of AI involves agents embedded in every enterprise workflow, where data is frequently transferred between systems and agents. This increasing interconnectivity adds layers of complexity to managing data security and privacy. Securing agent-to-agent interactions will become critical as AI agents handle more data autonomously. The interconnected nature of AI systems necessitates advanced privacy management strategies to address complex data flows. Organizations will also need rigorous onboarding protocols for both internal and external AI agents to ensure compliance with privacy principles.
Furthermore, embedding zero trust and privacy-by-design principles from the outset will be essential in building resilient AI systems. Monitoring user activity and implementing behavior analytics will also become key components of future data security strategies to detect and respond to internal threats swiftly.
Products like Protecto are designed to address these evolving challenges, helping companies manage AI agents securely and maintain trust in their data systems.
Image credit: md3d/depositphotos.com