How failure to identify AI risks can lead to unexpected legal liability [Q&A]

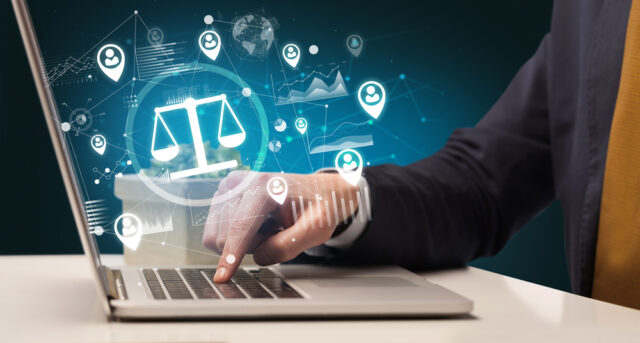
Use of generative AI is becoming more common, but this comes with a multitude of inherent risks, security and data privacy being the most immediate. Managing these risks may seem daunting, however, there is a path to navigate through them, but first you have to identify what they are.
We talked to Robert W. Taylor, Of Counsel with Carstens, Allen & Gourley, LLP to discuss how a failure to identify all the relevant risks can leave businesses open to to unexpected legal liabilities.
BN: What are the risks involved with generative AI deployments?
RWT: The most prevalent AI deployments are now use cases involving generative AI (GenAI) with a multitude of inherent risks. Security and data privacy are the most immediate risks people reference, but there are many more, including the technology hallucinating or creating errors or bias. MIT has identified 777 categories of risk with AI. Others I work with have identified a higher number of risk categories in security alone. However, these are the potential types of risks that can arise. They don't necessarily arise in every use case.
A complicating factor is that relevant risks and variables vary from use case to use case and are highly dependent upon the facts of each deployment. Yet another complication is that these risks and relative degree of risk are constantly changing throughout the lifecycle of a solution deployment.
A lot of the inherent risks are unintended consequences that are not readily apparent at first blush.
BN: What can businesses do to mitigate these risks?
RWT: There is a path to navigate through these risks, but first you must identify what the risks are in order to mitigate them. Failure to identify all the relevant risks can result in unexpected legal liability.
The only way to get a handle on the relevant risks is to do a holistic legal risk assessment which involves an in-depth examination of the AI solution and how it works in a given use case. An AI solution can have different risk profiles depending upon how it is used for a particular use case.
Injecting AI into your solutions and operations has a cascading effect throughout the organization. Just as there are many risk categories, so, too, are there many risk mitigation measures that can be adopted. Examples include updating customer contracts to address AI liability, providing effective notices of AI-generated content in user interfaces, pop-up notices about user requirements to be the human in the loop and validating outputs for accuracy.
To be able to oversee a GenAI technology and determine if it is going rogue, you need to know what you're asking the LLM to do and whether the GenAI outputs are correct or not. This is where human oversight comes in.
Recent legal challenges are instructive here. In a case brought against Air Canada, the airline was found liable for using a chatbot that hallucinated and told a customer it could buy a ticket and get a bereavement credit later. While having a human in the loop may not be technically feasible in a chat situation while the chat is occurring, as it would defeat the purpose of using a chatbot, you can certainly have a human in the loop immediately after the fact, perhaps on a sampling basis, to check the chatbot to see if it's hallucinating so that it can be quickly detected to reach out to affected users and also tweak the solution to prevent (hopefully) such hallucinations happening again.
Companies need to conduct a holistic legal risk assessment by a lawyer who understands AI and technology, who can work with the cross-functional teams to identify the risks, come up with mitigation options for each risk, and develop a compliance plan tailored to the use case. The firm has created its AI Triage Center to handle these issues and advise clients appropriately no matter where they are on their AI journey.
BN: Do you have examples of how specific GenAI deployments ended up having hidden risks or high risks that didn't appear at first sight?
RWT: Chatbots are a great example of this. They are being deployed widely across all industries for many purposes. In doing my assessments, I often am told that 'a chatbot is a chatbot' and that they're all the same and harmless. That's a myth. The risk profile is highly dependent upon the use case. For example, I see a lot of companies using chatbots in the HR context to answer questions about company policies and procedures.
The use of AI in talent and HR is generally a high-risk activity. The chatbot example I give here is what if an employee asks a chatbot, "When does open enrollment close?" The chatbot hallucinates and says it closes on December 31, when in reality it closes on October 31. The employee waits until New Year's Eve to sign up for benefits only to find that he or she missed the window. As a result, the employee doesn't get health insurance the next year. Shortly thereafter the employee is diagnosed with cancer and runs up $500,000 in medical bills. Who is on the hook for that? Arguably the employer and potentially the vendor of the chatbot.
Another example of hidden risk is another talent and HR use case. Companies are widely leveraging AI solutions to help with recruiting, filtering resumes and finding top candidates for a given job opening. In assessing one of these AI recruiting solutions, I asked the CTO leading the development efforts about how the solution was designed to mitigate bias. He responded that it was an impossibility because none of the personal data such as gender, name, race, etc. was ingested by the solution. All that data which could drive bias was stripped out. I went through the exercise with him and explained that you're training the solution on years of historical hiring data, resumes, job offers, rejections, etc. The solution learns that people from a certain university never receive a job offer or an interview. The solution draws an association and learns that the particular university is disfavored and therefore de-ranks candidates from that school, which means future applicants from that university don't make the top cut to be in the running for a job opening. That university happens to be Howard University which has a predominantly Black or African American student population. That's how an AI solution can create bias even though no race or ethnicity information is ingested.
BN: From a legal standpoint, are you seeing developers or users of GenAI solutions being sued? Or both?
RWT: End users and developers have potential liabilities and are being sued for many reasons which all tie back to the risks that come with AI. A recent class action was brought against Patagonia which deployed a third-party AI solution in its call center that was listening in on customer calls, transcribing them and doing analytics. The foundation of that case hinges on the fact that Patagonia did not provide notice of this use and/or obtain consent from the callers. Other companies have been sued over their AI chatbots that hallucinated. Many other cases are likely to follow as we enter the second phase of AI litigation focused on users and developers rather than the creators of the LLM models. We’re also seeing a lot of activity by various state attorneys general that are opening investigations into both developers and deployers of AI solutions. This is to be expected as AI is a hot topic, and we usually see government agencies looking active to address 'bad actors' to justify their existence.
BN: How do you see this space evolving in terms of risks and potential lawsuits with the introduction of new technologies like DeepSeek?
RWT: Time will tell on this. I think companies signing up directly with DeepSeek should be wary given all the concerns about privacy and data being stored in China and the growing number of investigations by attorneys general and regulatory agencies around the world.
Image credit: ra2studio/depositphotos.com