Beyond detecting bots -- in the age of AI, it's all about intent

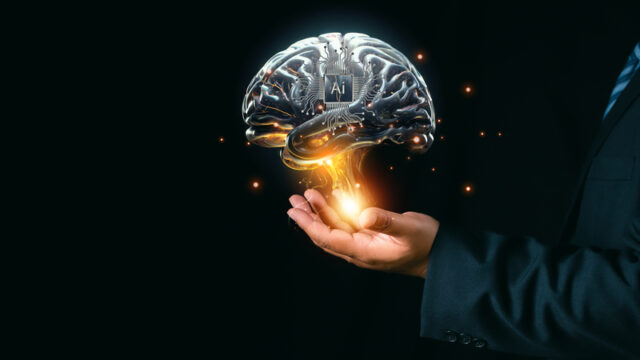
For the past two decades, cybersecurity teams have been laser focused on identifying ‘what’s bot -- and what’s not’. Over the past couple of years, this focus has shifted slightly, with security teams dedicating most of their attention to the most sophisticated bots.
New developments in AI over the past year have added a new level of complexity, with the emergence of beneficial business bots, like sophisticated AI agents, complicating what was once a binary task of differentiating bot from human.
In an era where AI can be used for good, and human activity can be malicious, it’s no longer enough for cybersecurity teams just to detect and block automated activity. The development of these hyper sophisticated, positive AIs means security teams must focus not only on ‘bot or not’, but the intent behind the AI.
How bot lines are blurring
In the past, there has typically been a clear distinction between good and bad bots. ‘Good automation’ has behaved in a simple and straightforward way, for instance basic web crawlers that followed predictable patterns; RSS feed readers that checked websites for updates; and simple monitoring bots that pinged websites at regular intervals to check they were operational.
But today, legitimate business bots -- like SEO tools and AI agents -- are designed to be as complex as a human, and use many of the same technical approaches cybersecurity teams have seen before in malicious bots. For instance, AI agents are often able to solve CAPTCHAs, mimic human browsing patterns, and interact with cookies in a way that a human would.
Meanwhile, as good and bad bots become more sophisticated, so do human attackers. These cybercriminals have a wealth of tactics at their disposal, both with and without the use of bots.
Bad actors don’t need bots to wreak havoc: they can take advantage of social engineering techniques to find the cracks in organizations’ cybersecurity strategies and exploit individuals. Ten years ago, a phishing email might have been laughably obvious, but attackers have evolved and learnt from their mistakes, and these messages are now convincing enough to trick even savvy employees and individuals.
And just because a human is accessing your service doesn’t mean they are not aided by a bot; historically, there has often been a ‘human in the loop’ to carry out authentication processes like CAPTCHA and to make decisions. Today, agentic AI makes its own decisions, as an agent of the legitimate or illegitimate human operating it.
Just because a user looks human doesn’t mean they have good intentions, and just because activity is automated doesn’t mean it’s bad. So the real question isn’t who (or what) is visiting your website, but why. Is the intent legitimate, like a search engine crawling for indexing? Or is it malicious, like an AI bot trying to scrape data or break into accounts?
Identifying intent
Shifting to intent-based security measures means overhauling the way organizations look at cybersecurity threats. Let’s look at three opportunities where organizations can test intent rather than simply blocking automated activity.
The first approach is looking at advanced behavioral analytics. These systems track patterns across multiple sessions to decipher positive and negative intent. For instance, a legitimate price comparison scraper bot might access 100 product pages at regular intervals twice daily, while a malicious data scraper bot might visit 10,000 pages randomly throughout the day, each time using a different IP address.
By looking at these patterns over time, cybersecurity teams can differentiate between similar technical actions with different intents.
Once your organization has established what normal behaviors look like, it becomes easier to detect and flag anomalies. AI-powered anomaly detection ‘learns’ what is normal behavior for different segments. Let’s take an online banking service as an example.
With anomaly detection, the organization might spot that an authenticated user is suddenly accessing areas of the account portal they’ve never visited before, and attempting to transfer funds to different accounts. Even though this user has valid credentials, with anomaly detection this kind of behavior would be picked up as out of the ordinary -- and transactions could be blocked in time to prevent disaster.
Another approach is to assess how much of your system’s computing resources each user or session is consuming, but basing this on flexible, context-specific limits -- rather than setting a ‘one-size-fits-all’ limit. For instance, an e-commerce retailer trying to prevent scalper bot activity might currently have static rate limits in place to block any IP address that attempts to make more than 100 requests a minute.
This kind of system might work well during normal shopping periods, but during peak shopping dates -- like Black Friday or Boxing Day -- shoppers often scan through lots of different items, and they might be blocked as a result. Meanwhile, advanced scalper bots might be able to stay under the threshold and manage to snap up limited products to sell on at inflated prices.
Here’s where dynamic resource monitoring comes in. A legitimate user will typically explore different options, consider their choices, and sometimes abandon their cart, which means they’re using a varied amount of resources. But scalper bots (even sophisticated ones) will often focus on specific, high-value product pages, without clicking around the website.
Plus, if a bad actor is using multiple scalper bots, the system should be able to spot that tens or hundreds of apparently ‘different’ users are all following the same resource consumption patterns. With intent analysis, this kind of activity will be flagged and stopped, protecting the retailer’s reputation among genuine customers.
Friend or foe?
A binary approach to human and bot activity no longer goes far enough for organizations to protect themselves and their customers. Humans aren’t necessarily friends, and bots aren’t necessarily foes. This is why we need security that’s just as smart as AI - bad intent requires better AI.
Businesses need security solutions that are just as adaptive as the threats they’re facing - ones that can tell the difference between good and bad AI in real time. Unlike traditional security approaches that need to be updated every time a new attack technique emerges, an intent-based system can detect even new threats based on unusual behavior.
This is the only approach that is able to keep up with the evolving sophistication of today’s attackers, both humans and bots.
Image Credit: Wanniwat Roumruk / Dreamstime.com
Benjamin Barrier is co-founder and Chief Strategy Officer at DataDome