How AI can help prevent 'catastrophic forgetting' of malware data

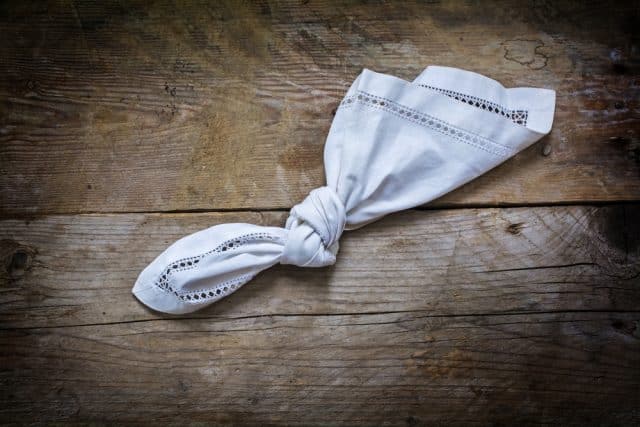
With large numbers of new samples appearing every day the old signature-based methods of malware detection have become unwieldy.
AI can learn from millions of samples, but if it uses all samples for optimum detection that means slower learning and updates. The alternative is to use only select samples to keep up with the rate of change of malware, but this runs the risk of 'catastrophic forgetting ' of older patterns.
The SophosAI team have been evaluating these options to see if it's possible to have a fine-tuning model that can keep up with the evolving threat landscape without harming performance, and researcher Hillary Sanders has published the findings in a new blog post.
The team tried out various learning methods: data rehearsal, based on a mix of old and new samples; learning rate, where the model adjusts based on how much data it sees; and elastic weight consolidation (EWC) which uses an old model to 'remind' the new one if it starts to forget.
All models performed better on older samples than new ones, but both the EWC and learning-rate approaches remove the need and cost of maintaining older data. Their future performance (using new data) is stronger than that achieved using the data-rehearsal technique, but data rehearsal is better on old data.
Sanders concludes, "In the malware detection game, being able to remember the past is almost as important as being able to predict the future. This must be balanced against the cost and speed of updating your model with new information. Data-rehearsal is a simple and effective way to protect the model's ability to detect old malware while significantly increasing the pace at which you can update and release new models."
You can read more on the SophosAI blog.
Image credit: fermate/depositphotos.com