Why hybrid is the future of AI [Q&A]

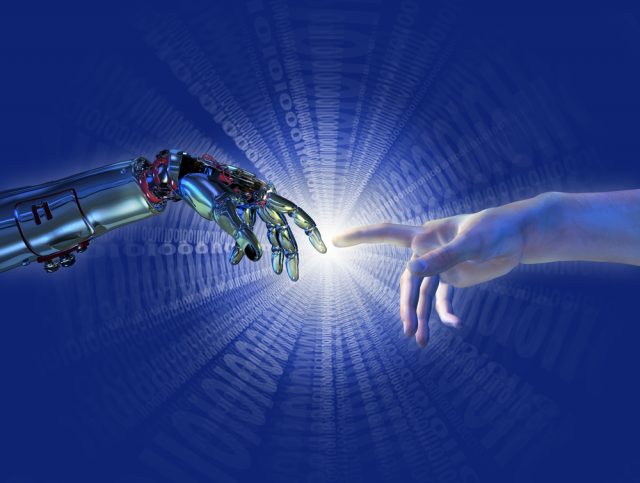
Artificial intelligence, along with machine learning, deep learning and natural language understanding are increasingly being combined to create a hybrid approach.
Harnessing a range of techniques in this way helps data scientists to produce the best results. We spoke to Luca Scagliarini, chief product officer at expert.ai to find out more about how this approach can work and why we can expect to see it becoming more common.
BN: What exactly is hybrid AI?
LS: Hybrid AI combines different techniques to improve overall results and better tackle complex cognitive problems. Hybrid AI is an increasingly popular approach for natural language understanding (NLU) and natural language processing (NLP). Bringing together the best of AI-based knowledge or symbolic AI and learning models (machine learning) is the most effective way to unlock the value of unstructured language data with the accuracy, speed and scale required by today's businesses.
ML can be very powerful, because it finds patterns and draws inferences from data. But this poses some problems, because if ML is trained on biased or incomplete data, its conclusions will share that inaccuracies and bias. For example, Amazon famously had to scrap an ML-based recruiting tool that had been in development for over a year because, since it had been trained on ten years of resumes that mostly came from men, the tool had taught itself that men were superior to women and made its choices accordingly.
Symbolic AI, on the other hand, is rule-based, with code that is leverages the intrinsic knowledge of the subject matter experts. So the symbolic approach doesn't require the considerable amount of training data that ML does and it's energy efficient and explainable.
Hybrid AI, combining symbolic and ML, has the advantage of leveraging the strengths of each approach while remaining explainable. ML can target pieces of a problem where explainability isn't necessary, while symbolic AI can reach conclusions and make decisions via a transparent process.
BN: Why is the hybrid approach to AI becoming more common?
LS: The capability to understand language remains one of the hardest challenges in AI. Machine learning is capable of supporting specific language-intensive applications; however, it is limited from delivering optimal results. A knowledge-based or symbolic approach relies on a knowledge graph to embed knowledge and is structured in a way that is similar to how we build our own knowledge. Moreover, it offers explainable AI as its outcomes are tied to explicit representations of knowledge.
The inability to explain how AI reaches its conclusions poses a real problem for businesses, especially if they work in regulated industries. With traditional ML, data goes in, results come out, and no one has any idea how it came to its conclusions. It's impossible to identify what causes errors. The only remedy is to retrain the entire system.
For example, if a lender uses ML to determine whether someone qualifies for a mortgage, they’d be hard pressed to defend themselves if they were accused of unlawful bias in their lending practices.
Plus, very soon, explainability may be required by state regulations. The EU has proposed AI regulations that, if enacted, would mandate that AI used for high-risk applications be 'sufficiently transparent to enable users to understand and control how the high-risk AI system produces its output.' That phrase 'high-risk' covers anything that could have a harmful impact on fundamental rights guaranteed in the Charter of Fundamental Rights of the European Union, which includes discrimination on the basis of sex, race and ethnicity.
Hybrid AI leveraging a knowledge-based approach enables AI to be explainable, which enables organizations to explain how the AI came to its conclusions and to correct any errors that arise.
BN: Can hybrid free up data scientists from tedious tasks like labeling data?
LS: Absolutely. Here's an example. An insurer with a large number of medical claims wants to use NLP to automate coding so that AI detects and labels the affected body parts in an accident on the claim. First, the insurer needs to make sure the AI is using current terminology, and ML is perfect for automating the understanding and mapping of the appropriate terms. There's no need for the process to be explainable because we already understand the desired outcome and can check whether the output is correct using a dictionary.
The system could then capture the data in the claims and normalize it. If the left knee is injured in an accident, symbolic AI can detect all synonyms, understand the context and apply a code of the body part involved. It's transparent because the insurer can see where it's coded with a snippet from the original report. There's a massive efficiency gain, but ultimately, humans are still making the final decision on the claim.
BN: Does this aid in understanding why a system is behaving in a particular way?
LS: Yes, definitely. If there are errors, symbolic AI provides a clear process to backtrack to identify the source of the mistake.
BN: What are some of the practical applications for this approach?
LS: There are many, especially in regulated industries where companies are leery of AI because it has previously been an opaque process. Insurers can use NLP that leverages hybrid AI to automate claims processing, banks can use it to help lending officers better assess risk, and information service providers can use it to pull relevant information from financial reports for more sophisticated analysis. Really, the sky's the limit.
Image credit: AlienCat/depositphotos.com