Behavioral analytics and why it's important to threat detection and response [Q&A]

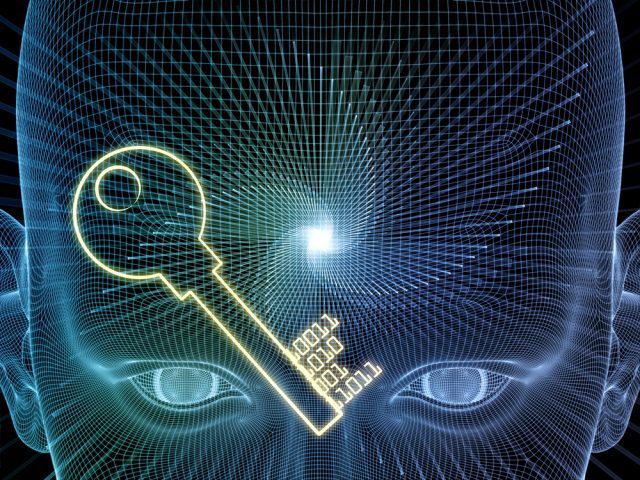
Traditional rule-based security techniques centered on malware signatures and perimeter protection are increasingly unable to cope with the latest, more sophisticated threats.
Taking a more behavior-based approach to spotting unusual or risky activity offers a solution, but what is required to make it work? We spoke to Sanjay Raja, VP of product marketing and solutions at cybersecurity specialist Gurucul, to find out.
BN: What is behavior analytics and why is it important in threat detection?
SR: Behavioral risk analysis examines network, application, cloud, user, and device activity for behavior that is both unusual and high-risk. This requires machine learning (ML) models that baseline normal behavior and look for anomalies. But not all unusual activities are risky. For example, consider a marketing employee accessing marketing materials from a SharePoint drive for the first time in several months. This is unusual compared to her normal behavior, but likely relatively low risk. But that same employee accessing code repositories from an unfamiliar location in the middle of the night when most employees are offline is much riskier and should be flagged.
When done successfully, behavioral risk analysis can improve efficiency, reduce false positives, and detect insider threats and zero-day attacks that other threat detection methods cannot. As a side benefit, the ML analysis involved can also produce valuable data on how systems and devices are used (for example, looking at the normal usage patterns for a system or a set of devices could let the IT team know the best time to shut it down for updates).
BN: What makes for good behavior analytics software?
SR: It's the technology behind the software that really makes it effective. A good behavioral analytics system will leverage true ML to detect and adapt to both known and unknown threats by conducting a risk analysis.
Conducting risk analysis involves determining the risk level of behaviors, which requires gathering a large amount of contextual data (usually into a data lake), correlating and linking that data to unique users and entities, running behavior analytics powered by ML, calculating a risk score based on that data, looking at an anomaly in light of that risk score, and prioritizing it accordingly. This helps to reduce false positives (behavior that is unusual, but low risk often triggers a false positive alert in less sophisticated solutions). This contextual information is the key to identifying what behaviors are risky or not. Contextual data might include relevant information to an incident such as events, network segments, assets or accounts involved. This contextual data is then sent to security teams to give context when further analyzing a detected threat.
BN: What are the differences between rule-based and machine learning in behavior analytics?
SR: Although rule-based and machine learning behavioral analytics may seem similar on the surface, they actually function very differently. Rule-based detection is often sold as AI or ML but isn't true AI or ML at all. Rule-based detection is essentially a flowchart that goes through a preset series of steps or tests (inputs) regardless of context and generates an alert (or output) if predetermined criteria are met. Machine learning engines will take context into account and assess how risky and how unusual a certain behavior is. For example, machine learning engines use baselines, peer group analytics, and anomaly detection to identify unusual behavior, like users accessing the network from unrecognized IP addresses, users downloading copious amounts of IP from sensitive document repositories not associated with their role, or server traffic from countries that the organization does not do business with.
The first key differences between these two detection engines is their ability to adapt to new variants of cyber-attacks. A rule-based detection system has a hard time detecting and adapting to new variants of malware and needs to be manually updated with every new variant that attacks the system. This results in slower behavioral analytics software that often will detect attacks too late. Whereas machine learning systems will detect new variants of malware by noticing suspicious network activities associated with it, even if the file itself is not known to be malicious, and flag security teams to further analyze the threat.
Another important difference between these two approaches is the need for human interaction. Rule-based detection systems need to be constantly manually updated by the vendor or security team. Depending on how responsive the vendor is or how experienced the security team is, this process can take days or even weeks. This in turn could result in a company’s data being further exploited, which creates heavy costs and more manual work for enterprises and their security teams. With machine learning, the need for human interaction and updating is greatly reduced because the system will automatically learn and adapt to new attacks and their variants.
BN: How has behavioral analytics technology changed in recent years and what improvements are on the horizon?
SR: Behavioral analytics technology has evolved significantly over the years with the implementation of true machine learning (moving past the rule-based approach) leveraging supervised, unsupervised and deep learning techniques. As malware became more advanced and tactics like code obfuscation became more common, rule-based systems have had a hard time adapting to the new malware landscape. As machine learning models have become more sophisticated, behavioral analytics has improved in tandem.
The use of ML has allowed security teams to detect different kinds of threats and reduce costs in ways that could never be done before. Behavioral risk analytics has great potential to make threat detection more efficient and keep organizations safer. Building robust ML analytics drawn from adequate input data will be key to the success of this approach over the next several years as this technology becomes more standard in next-generation security systems.
BN: What are the choices businesses have when choosing and implementing threat detection and what should they do to be successful?
SR: With all of the different types of threat detection available, it can be both overwhelming and confusing for businesses to find and choose the right security product that fits their needs, skill levels and budget. One of the best things that a CISO can do is define their security needs and communicate them clearly with the security team.
When evaluating threat detection solutions from vendors, businesses should ask important questions such as:
- Will my team have to do a lot of manual correlation, and how are they able to accomplish that with events that span weeks or even months?
- Will my team have to search through multiple tools and put together context on their own to see patterns that will help formulate a better response when working with other IT teams?
- How can my threat detection platform automate certain tasks and bring the right context to the forefront?
- How can it provide the necessary context that can help a less-experienced analyst learn over time and increasingly add value?
BN: What problems or roadblocks prevent organizations from using behavioral analytics successfully in threat detection programs?
SR: One major problem that hinders many organizations is not gathering enough data to feed threat detection tools. If these tools don’t have complete networking or device data, then security issues could slip past in those blind spots. Too little data also means that threat detection cannot be as precise or contextual, which in turn means more false positive alerts and more work for SOC analysts to investigate and respond to the actual threats. Organizations might be restricting their input data because they mistakenly believe that doing things like turning on NetFlow will slow down network performance. Others might have a threat detection solution that charges based on the volume of data it ingests, so they are limiting inputs to keep costs down. In addition, many rule-based threat detection solutions cannot ingest 'unstructured' data from sources like proprietary business applications, Industrial Control Systems, IoT devices, or healthcare devices because it is not in a format they recognize.
A second issue is the quality of the ML models that the behavioral analytics relies on. The more models a solution has, the more detailed each one can be. This means they'll be more accurate and the solution will cover a wider range of security threats overall. A robust behavioral analytics solution should have hundreds of ML models. Many solutions also have proprietary ML models that can't be verified or customized. This also creates roadblocks because the user cannot verify that the models are working as intended and cannot modify them to respond to new threats. A better option is to choose a vendor that offers open analytics so that companies can customize the vendor’s machine learning models or build their own.
Image Credit: agsandrew / depositphotos.com