The evolution of AI in the enterprise [Q&A]

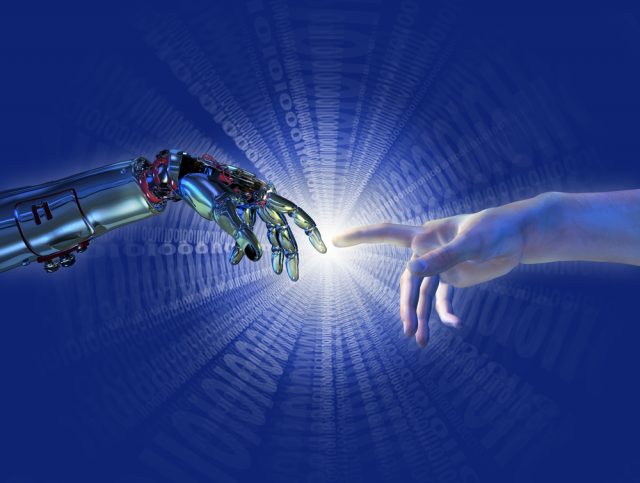
In the last year or so, AI has suddenly been the thing that everyone's talking about, thanks largely to ChatGPT. There's a good deal of discussion around where AI is headed in the future and the opportunities and threats it presents.
We spoke to Josh Tobin, CEO of Gantry, an AI observability tool for platform models, about the evolution of AI in the enterprise and how businesses can make sure they don't get left behind.
BN: It certainly seems that with ChatGPT AI has gone mainstream. Do you think we’re entering an era where AI will be truly ready for mass consumption/adoption?
JT: Yes, absolutely. Large language models are reaching a point of commoditization. Companies like OpenAI are reducing the barrier to entry and making these technologies widely accessible to any individual and organization. We're seeing an explosion of creativity from builders and founders. It seems like everyone from startups like Jasper to larger companies like Slack and Notion are using foundation models to augment their products.
And this is not entirely new. Startups and enterprises alike have been leveraging machine learning models as core components of their product for nearly a decade.
What has changed is not the adoption of AI, but its pace and visibility. Before, AI was behind the scenes -- producing research, contributing to analytics, and optimizing existing processes for enterprises. Now, ML is front and center in brand new product experiences. And that changes the expectations for ML teams. With foundation models, it's no longer about being great at modeling -- instead it's about building applications that solve problems for end users.
BN: Why has it taken so long for language models to reach the mainstream?
JT: It hasn't. The transformer model architecture was invented a little over five years ago, and OpenAI made the GPT-3 API generally available less than 18 months ago. Language models have reached the mainstream astoundingly quickly.
Why are they taking off now? OpenAI figured out a form factor that is more appealing to the average person. They have essentially consumerized it.
Just like the shift from mainframes to PCs, it was less about computing power and more about the form factor and affordability.
BN: Are most 'legacy' companies ready to embrace AI? Will they be able to compete with these companies that are born AI-first?
JT: AI is not something that you can tack onto an existing product and expect transformative results. Models by themselves are not products. They're a piece of technology that can be used to build great products. This means that for enterprises to truly realize the value of AI, they will need to rethink core assumptions about their business. This is not that different from the shift from on-prem to the cloud. Some companies will adapt; others will move slowly and get disrupted by new businesses that use the technology natively.
Legacy companies do have some significant advantages. They have more data and deeper knowledge of their customers. They also have the resources to build products that are useful even without AI, which helps bridge the gap while the AI doesn’t work well enough to be the star. If enterprises can become AI-first quickly enough, they stand to benefit from this technology as much or more than startups. But if history is a lesson, not all will be able to adapt quickly enough to avoid being disrupted by a leaner AI-native challenger.
BN: What can these companies do if they are to compete? What can they do to get on the AI train?
JT: First, they need to see the opportunity: AI has the potential to fundamentally change the way most products work. But to realize that change, companies may need to rethink their product and business model from the ground up.
Second, they'll need to face the reality: this technology is not a magic bullet. It doesn't 'just work', and building it requires different skills, tools, and mindsets than traditional software.
Finally, they need to recognize the risks: machine learning is an inherently risky technology. Models never work perfectly in all situations.
The above applies to any size organization. But there are specific considerations for incumbents versus startups.
For incumbents -- i.e., non-AI-native companies -- the most critical consideration is how this technology fits into their business. Many of the early applications in enterprise will look like thin wrappers around chat-based models. That's fine -- in fact it's a great idea to pick projects early on with a low timeline to impact. But in the long term, the most successful applications will be more closely tied to the unique user experience the companies are trying to create. Those types of experience only come through a mastery of your data and a deep understanding of the user experience you're trying to create.
For startups, the most important thing is to think about the product experience holistically, not just about the AI. Second, every AI startup needs to think about how to build a unique, long-term competitive advantage over every other company building on the same off-the-shelf models. In most cases, this will come by gaining data independence: owning the data about your use case and using it to personalize and customize your models.
Image credit: AlienCat/depositphotos.com