Data bias -- the hidden risk of AI and how to address it [Q&A]

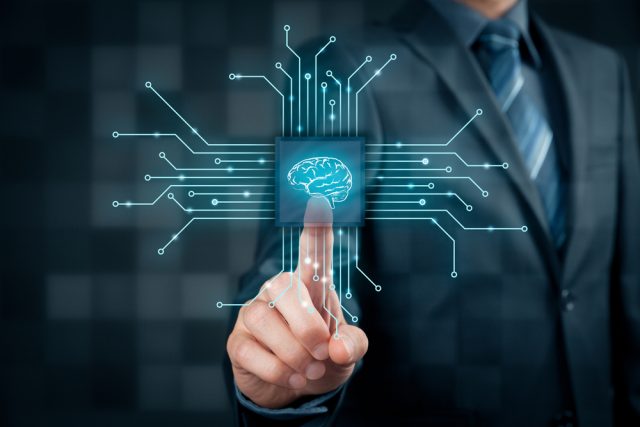
Artificial intelligence is generally only as good as the data that it's trained on. However, when data is collected and used in the training of machine learning models, the models inherit the bias of the people building them, producing unexpected and potentially harmful outcomes.
We spoke to Matthieu Jonglez, VP, technology at Progress, to discuss the company's recent research around this topic and what organizations can do to reduce bias.
BN: What is data bias and what are the key reasons for it to develop?
MJ: When it comes to artificial intelligence (AI) and machine learning (ML), the algorithms are only as good as the data used to create them. If data sets are flawed, or biased, incorrect assumptions will be part of every resulting decision. While data bias is not a new concept in business, understanding the problem and its solutions is paramount for the future of business. Some businesses may be aware of bias to some extent, while a certain amount of bias is unconscious bias, which can lie undetected -- either way its impact on the organization, its workers and on society can be significant.
Data bias happens when human creators inject decision biases into systems by training them using biased data, or with rules that intrinsically reflect their personal biases. Biased data can include flawed data sets, blind assumptions, automated testing protocols that are not appropriately inclusive, and models that wrongly discriminate against under-represented groups.
When data is collected and used in the training of machine learning models, the models can often inherit the cultural and personal bias of those people building them, producing unexpected and potentially harmful outcomes. Other bias areas include user experiences that don't meet W3C accessibility standard, which is considered a form of bias against those with disabilities. It's all too easy for unconscious bias to creep in at any point during the AI and app development lifecycle.
BN: What's the current state of data bias in business?
MJ: To establish organizations' understanding of the risks of data bias and their readiness to take action, leading global software business, Progress, and research firm, Insight Avenue conducted a 2023 global survey of AI and ML data bias, Data Bias: The Hidden Risk of AI. This survey interviewed over 600 senior business and IT executives who use or plan to use AI and ML to support their decision making.
In terms of awareness, the research revealed that almost two-thirds of organizations (65 percent) believe there is currently data bias in their organization. Business professionals are aware of its importance -- with 77 percent admitting they understand the importance of mitigating data bias and believe it is prevalent within their organization yet struggle with how to address it effectively.
The biggest barriers to addressing the issue are identified as lack of awareness of potential biases, understanding how to identify bias as well as the lack of available expert resources, such as having access to data scientists. Since only 13 percent are taking steps to address it and have an ongoing evaluation process, there’s a clear need for education and action to address the current inertia.
The situation is likely to get worse -- as 78 percent of business and IT decision makers believe data bias will become a bigger concern as AI/ML use increases, and 66 percent of organizations anticipate becoming more reliant on AI/ML decision making in the coming years.
BN: In what ways can data bias impact business operations and decision-making?
MJ: The impact on business operations and decision making can be significant -- from governance and loss of customer trust to financial implications and potential legal and ethical exposure. The types of decisions impacted included finance, IT/digital, operations and customer acquisition. Moderate concerns included unfair stereotyping and negative impacts to inclusion and diversity efforts. When data bias goes ignored, the greater the chances of significant damage to the business, affecting security and governance, revenue, and reputational damage.
There are also profound consequences experienced by victims of data bias, including those who suffer adverse outcomes resulting from intrinsically biased AI algorithms. Beyond this, 76 percent of organizations themselves believe there could be wider societal impacts if enterprises were collectively unable to adequately address data bias.
BN: What are the benefits and the biggest barriers for businesses to address data bias?
MJ: Respondents do see great benefits in working to address data bias. This includes minimizing risk, making better decisions, advancing market opportunities, becoming an attractive employer for data scientists, and improving company reputation.
However, there is a lack of understanding around the training, processes and technology needed to tackle data bias successfully. In fact, 51 percent consider lack of awareness and understating of bias as a barrier to addressing it. For the 77 percent that believe they need to be doing more to address data bias, many of these may not know where to start in addressing it. In fact, only nine percent mentioned not seeing data bias as an issue, meaning inaction is more due to planning and execution than a failure to recognize the imminent threat bias presents.
Lack of skills also ranks a top barrier for organizations, with 31 percent citing lack of expert resources, such as having access to data scientists, as a challenge.
BN: What can organizations do to identify, address and mitigate data bias effectively?
MJ: Only a comprehensive approach that combines people, tools, training and ongoing policy vigilance will ensure data bias is eradicated from AI/ML practices. Organizations themselves identified tech/tools, training and strategy/vision as the most urgent focuses for combatting data bias.
Some fundamental considerations for organizations to get started with reducing data bias include:
- Creating a robust data bias policy -- One of the first steps should be to appoint an effective leader who can take a holistic view of data bias across the organization and drive policies for change. In fact, 76 percent of respondents agreed that data bias was best tackled centrally across the organization rather than in siloed departments. A clear majority (39 percent) named the Chief Information Officer/ Chief Technology Officer best equipped to own data bias initiatives, but a Chief Data Officer or COO would make equally effective leaders.
- Building transparency and traceability into AI use -- AI needs unbiased data to deliver unbiased results. Achieving this requires an agile, transparent, rules-based data platform where data can be ingested, harmonized and curated for the AI tool. Transparency is the antidote to bias. Data lineage features allow the human expert to track any changes made to the data, including back to the moment humans introduced bias.
- Developing mechanisms to identify and measure bias -- For those working to combat data bias, effective measures were found to include education and training; improved transparency and traceability of algorithms and data; more time spent model training, building and evaluation; and using tools to help source bias within data sets. Only a continuous commitment to assessment and removal will ensure bias doesn't seep in over time.
Other key focus areas should include training, hiring and team diversity. For AI to be sustainable over time, the pool of those developing these algorithms must become more diverse, across the racial and gender spectrums, those with less-advanced degrees and those from a broader cross-section of professions and backgrounds.
At a tech level, every touchpoint within the entire tech or development stack must factor in the reality of data bias. This should include the entire data selection and preparation process, business logic development and analytical models, testing and results analysis.
BN: What are the implications of data bias on the future of business?
MJ: Data bias is a problem that's set to escalate, as 66 percent of organizations anticipate becoming more reliant on AI/ML decision making. As AI/ML use increases, more data scientists, practitioners, and programmers will dive into datasets and produce ever-more algorithms. As our world becomes increasingly reliant on machines to make life-impacting decisions, it's up to those leading these efforts to ensure their work is a force for good.
Organizations must start by taking steps to understand the overall situation of data bias in their industry sector and specifically within their organization so that they can address it. Eliminating data bias will require a perfect combination of technology, training and practices to prevent it from entering the development process.
On a positive note, organizations are aware it's a problem that needs addressing. Taking some proactive measures to address and mitigate data bias effectively, as part of implementing a sound digital ethics strategy, is the way for leaders to demonstrate a responsible approach to AI use.
Image credit: Jirsak/depositphotos.com