How AI and vector search are transforming analytics [Q&A]

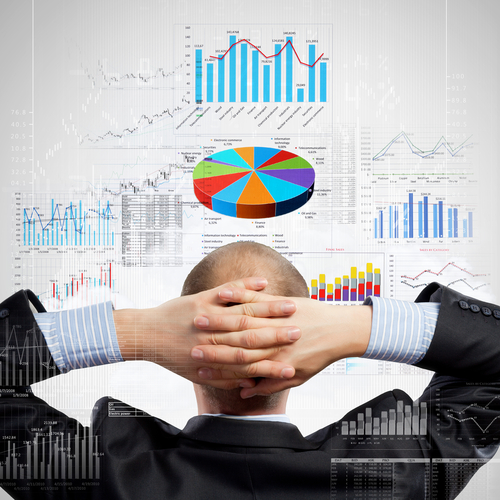
Organizations have more data than ever, but unlocking the information it contains in order to make decisions can be a challenge.
The marriage of of real-time analytics and AI with vector search is a potential game changer for any business that has large amounts of data to crunch. We spoke to Rockset CEO and co-founder Venkat Venkataramani to find out more.
BN: Why is vector search, fueled by AI and machine learning, having a moment now?
VV: Organizations continue to accumulate large quantities of unstructured data, ranging from text documents to multimedia content to machine and sensor data, with estimates showing it represents 80 percent of all generated data. However, only a small fraction of unstructured data is leveraged to extract valuable insights, power decision-making and create immersive experiences. Understanding how to take advantage of this data has remained challenging and costly, requiring technical depth and domain expertise, leaving it largely underutilized.
With the evolution of AI, neural networks, and large language models, organizations can easily transform unstructured data into embeddings, commonly represented as vectors. Vector search operates across these vectors to identify patterns and quantify similarities between components of the underlying unstructured data.
BN: What applications use vector search?
VV: Historically, search experiences primarily relied on keyword search, involving manually tagging data to identify and deliver relevant results, a process that requires a large number of steps. With vector search, users can leverage text embeddings to capture semantic associations across words, phrases, and sentences to power more robust search experiences.
Vector search has a wide range of applications across personalization and recommendations engines, anomaly detection and predictive maintenance. From powering personalization on e-commerce websites to identifying anomalous activity based on their similarities (and differences) to past activity, without the need to build complex and hard-to-maintain rules-based algorithms or manually configure text tokenizers or analyzers, search has become easier than ever. The possibilities for vector search are endless.
BN: What is the role of real-time data, analytics and vector search in AI/machine learning applications?
VV: The world is moving from batch to real-time, so that they can operate their businesses more efficiently. Banks monitor risk in real-time, insurance companies track hundreds of real-time factors while generating insurance policy quotes, and airlines are tracking all airport operations in real-time to ensure on-time departure of flights.
In addition to real-time reporting, monitoring, and anomaly detection, these businesses also want to do real-time predictions so that their teams can be alerted before something goes wrong. Sophisticated AI and machine learning techniques are what helps businesses make this transition.
BN: What are some common technical considerations when launching real-time AI applications in production?
VV: It is really important for real-time AI applications to incorporate best practices from both the world of distributed systems in terms of scalability and infrastructure, and the world of AI and machine learning in terms of model training and deployment.
Scalability: Ensure that your AI application can handle a large volume of real-time data and scale to meet increasing demand. This may involve designing a distributed system architecture, or utilizing cloud services that are built for real-time. Real-time applications require low latency to provide timely responses and establish a reliable and efficient data ingestion pipeline to collect and preprocess data in real-time. This may involve integrating with streaming frameworks like Apache Kafka and using real-time analytical databases, such as Rockset.
Infrastructure: Consider the hardware and software infrastructure needed to support your real-time AI application. This may involve selecting high-performance servers, GPUs, or specialized hardware accelerators to handle the computational requirements of real-time AI processing. Compute-compute separation, which isolates different workloads on the same shared data, is a must have for scaling real-time applications reliably in the cloud.
Model training, deployment and feedback loops: Real-time AI applications can benefit from feedback loops that allow continuous learning and model improvement. Establish mechanisms to collect user feedback and integrate it into the model retraining process to enhance performance over time. Choose a suitable deployment strategy for your AI models. This could involve containerization with tools like Docker and container orchestration platforms like Kubernetes. Implementing model versioning and deployment pipelines can simplify the process of updating and rolling back models.
BN: What are your predictions for the future of search experiences, and the increasing role of AI and machine learning?
VV: Heavy influence of AI and machine learning will be foundational to how search experiences will be constructed in the future. Here are some of those examples:
- Personalized Search: Real-time AI and machine learning algorithms will continue to improve search engines' ability to understand user preferences and deliver personalized search results. Modern real-time analytical databases will allow one to seamlessly integrate behavioral data along with advanced AI/machine learning vectors to provide more relevant and tailored search experiences.
- Natural Language Processing (NLP): AI-powered search will become more conversational and better understand natural language queries. With advancements in NLP and natural language understanding (NLU), search engines will interpret user intent more accurately, enabling more precise search results. As a consequence, visual and voice-based search experiences will gain prominence. Voice assistants and smart speakers will continue to improve their ability to understand spoken queries, providing more convenient search interactions.
- Semantic Search: AI and machine learning will enhance search engines' ability to understand the meaning behind words and phrases, enabling semantic search. This approach considers the intent and context of the search query, resulting in more accurate and contextually relevant search results. AI algorithms will improve search engines' ability to interpret user context, such as location, time, and personal preferences. This contextual understanding will enable search engines to deliver more precise and timely search results tailored to the user's specific situation.
The future of search experiences will likely hold even more innovations and surprises as AI and machine learning continue to evolve.
Photo Credit: Sergey Nivens / Shutterstock