The role of data governance in developing AI [Q&A]

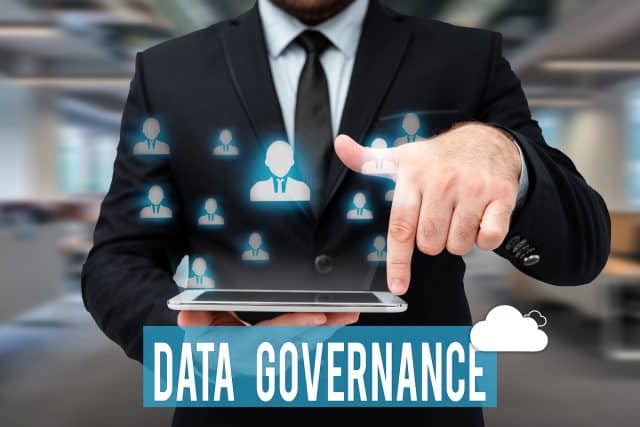
The term 'prompt engineer' has become a bit of a buzz word for future-of-work topics. What isn't discussed as much, and is arguably more important to AI models, is the role of the data governance architect.
Satish Jayanthi, CTO & co-founder of Coalesce believes that without good data governance, organizations will go nowhere in extracting value from AI and ML models. We talked to him to find out more.
BN: Why do you believe the role of a data governance architect is more crucial than that of a prompt engineer in the success of AI models?
SJ: I believe that both roles are important and have an impact on AI models but even the best prompt engineer knows that data governance impacts the quality of results -- that's why the role of a data governance architect is foundational in AI initiatives. It's analogous to ensuring the integrity of the building blocks before construction. This pre-processing step is critical; even the most advanced prompt engineers rely on the quality of underlying data to refine AI outputs.
While a prompt engineer, who designs and optimizes prompts for language models plays a significant role in determining the model's output quality, their work is contingent on the data already fed into the model. They can refine and guide the model's responses, but they cannot fix inherent flaws in the underlying data.
BN: What are the most significant challenges companies face in establishing effective data governance for AI and ML?
SJ: One of the biggest challenges is creating and fostering an organizational culture that appreciates and understands the value of data governance. It's a holistic approach that transcends IT departments. Additionally, the diverse nature of data sources and their inherent complexity present significant standardization challenges. Keeping up with the rapid evolution of AI and ML technologies also demands constant vigilance and adaptability in our governance strategies.
Additionally with the variety of structured and unstructured data, establishing standard procedures for data governance becomes complicated. It boils down to a balance of data access and security, ensuring that the right people have access to the right data while maintaining privacy.
BN: How does poor data governance affect the outcomes of AI and machine learning projects?
SJ: Inadequate data governance can significantly undermine AI and ML projects. The core issue lies in compromised data integrity, leading to biased or inaccurate AI outcomes. This can have serious repercussions, especially in critical sectors. Ensuring data accuracy and mitigating biases are not just technical necessities but ethical imperatives in today's AI-driven landscape.
Without standardized data quality checks, the same AI/ML models can produce different results under similar conditions. This inconsistency can be a significant hurdle in sectors where reproducibility is critical, like scientific research or legal analysis. Inconsistent data standards can also hinder the scalability of AI/ML solutions. If models are pulling from multiple data sets, the lack of standardization can lead to varying performance levels, making it difficult to generalize or scale solutions effectively.
BN: How does the 'garbage in, garbage out' concept apply to the training of artificial intelligence models?
SJ: The 'garbage in, garbage out' principle is particularly relevant when it comes to training artificial intelligence models. Essentially, this concept underlines the fundamental truth that the quality of the output produced by an AI model is directly dependent on the quality of the input data it receives.
In the context of AI training, if the input data is flawed, due to inaccuracies, biases, or incompleteness, the AI model will inherently learn from these flawed datasets. This results in the model developing biases, making inaccurate predictions, or failing to understand the nuances of the task it's designed for.
For instance, consider a ML model being trained for facial recognition. If the training dataset predominantly includes faces from a certain demographic and lacks diversity, the model will likely struggle to accurately recognize faces from underrepresented groups. This isn't just a theoretical concern; there have been real-world instances where such limitations have led to significant errors and biases in AI systems.
BN: What are some emerging trends in data governance that companies should be particularly aware of?
SJ: We are witnessing a surge in automated tools for data governance, which is a response to the overwhelming volume and complexity of data. Another significant trend is the emphasis on ethical AI, focusing on bias prevention and fairness. Moreover, the shift towards cloud-based data solutions is placing an increased emphasis on robust governance frameworks for distributed systems.
Biden’s executive order on AI regulations from last year is only the beginning, as AI and data governance will continue to be regulated to keep customers and businesses safe, even though the technology is advancing rapidly. This will force companies to collaborate with ethical committees, regulators, the government and external experts to ensure privacy is maintained.
The shift towards cloud-based data solutions is also reshaping data governance strategies. This transition requires robust governance frameworks that are capable of managing data across distributed systems. Cloud environments offer flexibility and scalability but also introduce challenges in terms of data security, privacy, and compliance, which need to be addressed through comprehensive governance policies.
BN: What strategies would you recommend for organizations to improve their data governance in preparation for AI and ML adoption?
SJ: To enhance data governance for AI and ML readiness, organizations should start by developing and implementing comprehensive data governance policies. These policies are critical as they establish the standards for data handling and ensure alignment with the organization's AI and ML objectives, while also adhering to regulatory compliance.
Building a data-conscious culture within the organization is essential. This involves not only training employees on the importance of data governance but also embedding a sense of responsibility for data integrity across all levels. Creating awareness and understanding about the impact of data on AI and ML outcomes is a key part of this cultural shift.
Continuous evaluation and refinement of governance strategies are necessary to keep pace with the evolving data landscape and technological advancements. This includes regular audits and assessments to identify areas for improvement and adaptability to new challenges and opportunities.
Image credit: nialowwa/depositphotos.com