Apps, analytics and AI: 4 common mistakes

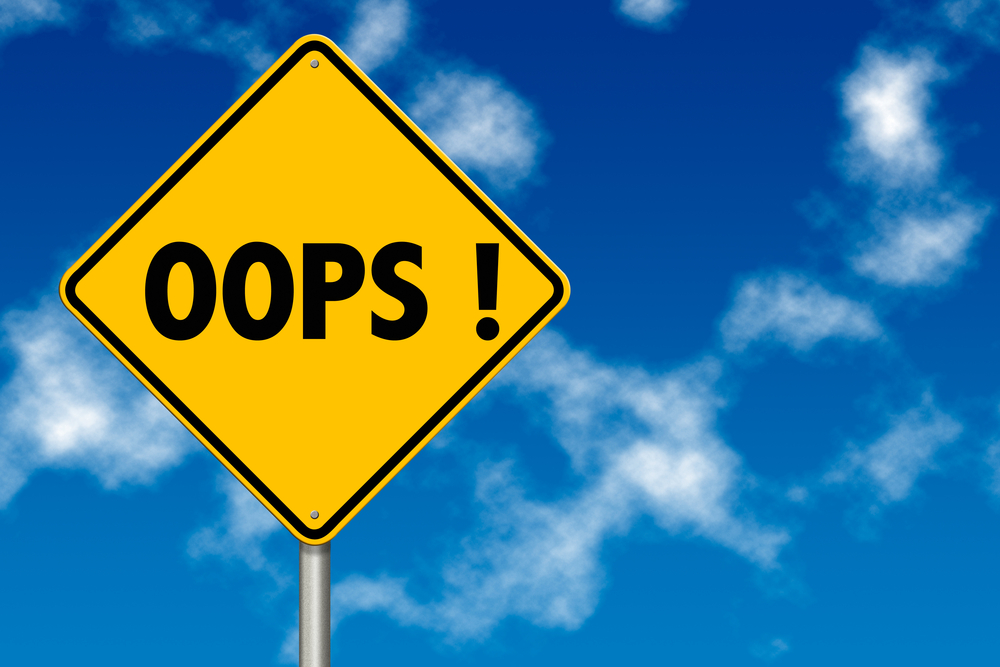
The app economy is big business. Apple’s App Store ecosystem alone generated a staggering $1.1 trillion in total billings and sales for developers in 2022. But as users demand more relevant and immediate experiences, often driven by AI, developers increasingly need competitive advantages to stand out.
Real-time analytics, supercharged by generative AI, can provide a critical edge by allowing developers to extract key insights and quickly adapt their apps to reflect changing user expectations. But only 17 percent of enterprises today have the ability to perform real-time analysis on large volumes of data, and adoption remains slow. Meanwhile, even when companies are able to perform real-time analytics, there are several common mistakes that can prevent them from reaping its full benefits:
- Too much focus on speed over accuracy and data quality
Timeliness is obviously critical to apps using real-time analytics. But speed shouldn’t come at any cost. The old adage “garbage in, garbage out” applies here: if a service draws on poor quality data, it will not deliver the intended outcomes. Outdated or incomplete datasets will only lead to inaccurate insights and erode customer trust in the application. Organizations should instead prioritize data validation checks and cleaning, as well as regular audits, to maintain data integrity and accurate results.
- Ignoring the importance of context
Real-time data requires broader context and correlation to help derive accurate insights. That’s why organizations must dig deeper to uncover the true relationship between variables. For example, a sudden spike in sales of an item may be due to increased consumer demand, macroeconomic conditions such as a shortage of complementary goods, climate-related indicators or perhaps promotional campaigns. Correlation does not imply causation.
- Failing to clearly define objectives
Analytics projects will rarely reap the desired rewards without specific, measurable goals. Organizations must therefore define clear objectives, such as improving customer retention by a certain amount within a set timeframe. This will help guide data collection and analysis efforts. Without clear goals, it’s difficult to identify actionable insights or measure success.
- Choosing the wrong tools
It’s important to remember that not all analytics tools are created equal, and an existing analytics tool may simply not have the power to handle real time data. It’s critical that organizations choose technologies tailor-made for real-time data processing and visualization. Failure to do so could lead to bottlenecks, latency and accuracy issues.
Getting it wrong and right
If organizations are able to successfully harness real-time data and couple it with AI, they can open the door to hyper-personalized and immediate “adaptive applications." These provide the kind of customized, dynamic and responsive experiences that customers are increasingly demanding, by adjusting behavior and features in real time based on factors such as user preferences, environmental conditions, data inputs and changing circumstances.
An adaptive retail app, for instance, might enable businesses and advertisers to offer the right products and services to the right target audience at the right time. Similarly, a booking app with adaptive functionality might be regularly updated based on real-time travel information, events and user history to suggest personalized journeys and deals.
Integrating generative AI with real-time analytics offers numerous additional benefits, including enhanced predictive capabilities, personalized user experiences and improved operational efficiency. This significantly enhances use cases ranging from fraud and anomaly detection to customer service and retail checkout experiences. By leveraging these technologies, businesses can gain deeper insights, respond faster to changes and deliver better products and services to their customers.
Likewise, there are potentially serious business consequences to getting real-time analytics wrong. Some 41 percent of enterprises claim they could go out of business within three years if their apps no longer meet user expectations. An even greater share (46 percent) believe they’ll lose out to the competition if this happens. Yet while these capabilities are already being used by mature, tech-focused businesses, the vast majority of organizations struggle to get hold of the right tools and know-how to leap barriers like siloed data systems.
Much of this comes down to an organization’s database architecture. A problem that has faced the database sector for decades is how to ensure real-time analytic results are immediately written back to the operational database and the applications it serves.
Things are on the up, though -- more modern databases can weave together both operational and analytical workloads, while ingesting and processing real-time data. Ideally, this is done in a single environment. This avoids moving data from databases to data warehouses, and eliminates the need for costly Extract-Transform-Load (ETL) processes in OLTP and OLAP systems, which can also introduce latency.
If app developers can avoid common mistakes, harness AI and modernize their database architecture, we could soon start to see a landscape where apps are more sophisticated and user-centric than ever.
Photo Credit: doomu/Shutterstock
Rahul Pradhan is VP Product and Strategy, Couchbase.