The challenges and opportunities of generative AI [Q&A]

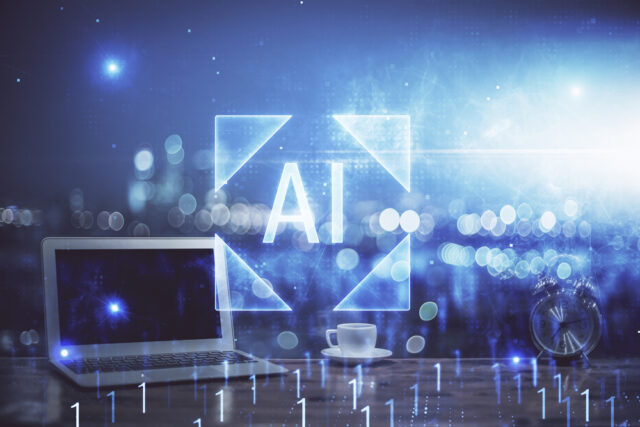
The promise of GenAI is undeniable, it offers transformative potential to streamline workflows, boost efficiencies, and deliver competitive advantage. Yet, for many organizations, the journey to implement AI is far from straightforward.
Obstacles typically fall into three categories: strategic, technological, and operational. We spoke with Dorian Selz, CEO and co-founder of Squirro, to explore these obstacles in more detail, as well as looking at some of the biggest misconceptions enterprises have when starting their GenAI journey.
BN: When companies start on their generative AI path, what are some of the most common misconceptions they have?
DS: One of the most typical misconceptions is that GenAI is easy to implement. Organizations underestimate the intricacies involved with the technology and what it takes to be successful with it.
Oftentimes businesses think GenAI is just a matter of using ChatGPT or deploying a chatbot. There's so much more involved that needs to be taken into account when starting on the GenAI path. Organizations must address several critical areas, including how they will protect sensitive data, comply with corporate and regulatory requirements, maintain stringent enterprise security, and scale as business needs require.
BN: How can businesses determine if they're AI-ready? What types of barriers do they encounter when trying to implement AI if they’re not?
DS: A clear sense of direction is the first step towards being AI-ready. Some of the questions that businesses should consider include: What is my approach to AI? What goals do I have for my company's GenAI efforts? Defined goals are essential for improving decision-making, streamlining operations, and spurring innovation.
This lack of strategic purview is one of the common roadblocks that businesses face -- they jump into GenAI without thoroughly considering the technology's potential for the company and having a well-defined use case or plan. As a result, they can't effectively assess its value or how it affects their overall revenue and net profit.
Organizations also encounter technological hurdles when they aren't AI-ready. These can be extremely difficult to overcome, causing companies to fail with their GenAI efforts more often than not.
To generate meaningful insights with GenAI, businesses need to ensure they have the technical capability to clean, validate and organize the unstructured data contained throughout their enterprise systems. Garbage in equals garbage out.
Additionally, businesses rarely have a single data access strategy in which all employees, from CEOs to the lowest-level employees, have the same access rights. Building a safe, permission-enabled GenAI system is extremely difficult and requires specialized knowledge that most companies don't have internally.
Also, from a technological perspective, it requires significant infrastructure and resources to scale AI solutions. Although a pilot project or prototype may appear promising, businesses frequently underestimate how complicated and expensive it will be to scale GenAI throughout the entire company.
Lastly, companies that are aiming to implement GenAI but who aren't operationally ready will encounter challenges with monitoring and maintaining the systems given the significant effort required to do so. They may run into issues with security and control over sensitive data, too.
BN: Should companies consider developing their own GenAI solutions given there are publicly available platforms?
DS: There are some companies that have the knowledge and resources needed to build their own GenAI solutions from square one. However, even with that foundation, many underestimate how complex it can be. While businesses may feel they have more control and customization opportunities with in-house GenAI development, these initiatives typically result in unforeseen expenses, technological difficulties, and scalability constraints.
For example, developing a GenAI application for a single use case is fairly straightforward, but scaling it to handle multiple use cases often means starting over each time. This results in rising development and maintenance costs. Even for businesses that have the necessary resources, scaling demands specialized knowledge and capabilities that most internal teams don’t possess.
BN: Are there specific challenges that can derail internal GenAI projects?
DS: Yes, and they are typically around scalability, compliance, and privacy and security.
Access control lists (ACLs) are essential for protecting sensitive data. However, integrating them into retrieval-augmented generation (RAG) applications (ones that amplify the performance of large language models (LLMs) by including additional data from internal or external sources) is very complicated. Unlike standard search, incorporating ACLs into vector search significantly boosts computational complexity, resulting in performance delays. This technical challenge often prevents in-house solutions from successfully scaling.
Additionally on the topic of scaling, internal GenAI projects are often not built to expand beyond one use case or handle incorporating millions of live documents across many repositories and supporting thousands of users.
With regards to complying with regulatory and corporate standards, GenAI solutions need to be developed with AI guardrails to ensure ethical and compliant outputs. Many GenAI proof of concepts (PoCs) that are built in-house aren’t able to fully meet stringent compliance requirements. This creates risks that can hinder large-scale deployment.
Finally, internally developed GenAI solutions often hit significant security roadblocks, including the ability to safeguard sensitive data, meet information security standards, and ensure security during enterprise systems integration. Organizations need specialized skills in addressing these issues, which many don't have.
BN: What advice would you offer to businesses who are looking for a pre-built GenAI solution?
DS: Seek out providers that have proven to deliver highly secure, compliant, and permission-enabled deployments at scale with accurate results, like Squirro. Platforms should come pre-configured to address common hurdles like security, compliance, and scalability, freeing internal staff to concentrate on innovation and core business objectives. If a business is in a regulated industry, proven successes in those are a must.
Organizations should also look for solutions that enable them to break down existing data siloes and streamline data integration across multiple repositories, as well as integrate seamlessly with existing systems. With new LLMs and technologies emerging, these platforms should also offer the flexibility to adapt to ensure businesses can continue operating most efficiently and rapidly address changing client needs to maintain a competitive advantage.
Image credit: peshkova/depositphotos.com