Data integration: Why it's essential for every successful business

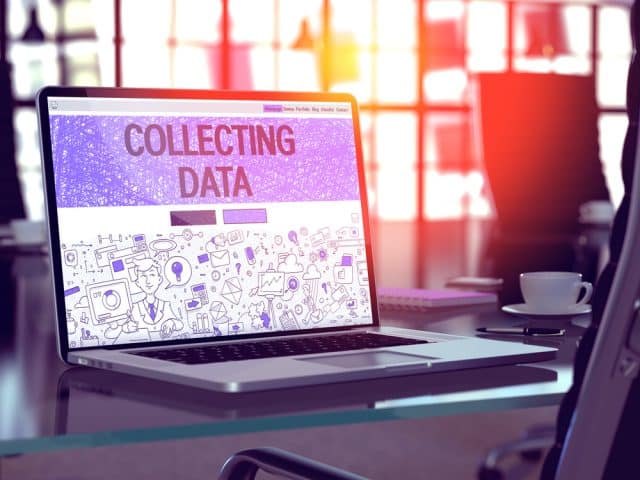
Ninety percent of the world's data was created in the past two years alone. Now, industry experts predict this volume of data will double in size every two years. To keep up with the rise in data volume, more companies are starting to transition from on-premises to cloud data storage.
Data integration is essential as businesses scale their operations, handle large amounts of data, and make their data more accessible. So, it's no surprise 81.7 percent of companies now have a mix of legacy and modern cloud technologies, highlighting the rapid transition to cloud data storage. Without effective integration, companies risk mismanaging their important data, leading to inaccurate reporting, tracking, and decision-making that could negatively impact their business.
Accessibility
Sixty-seven percent of enterprises rely on data integration to support current analytics and BI platforms. Due to this, it's critical companies have access to and control of their data 24/7. When companies integrate from on-premises to cloud storage, their data is located in one place and accessible to more individuals. They can easily import and export data from one application to another, allowing the information to be analyzed at micro and macro levels, resulting in improved operational insights. Additionally, enterprises also have control over security protocols and access to large quantities of data.
There's always been an urgent need for enterprise customers to analyze data, conduct reporting governance, and merge data between different applications to gain deeper insights. While these processes are available with on-premises applications, the cloud eliminates barriers and offers more room for data and access to critical information. With data integration, SaaS applications can act like on-premises applications, streamlining the whole process by saving time and allowing for data manipulation without corruption.
Automated data flow
Enterprises must be sure no errors occur during data integrations. However, sometimes mistakes still happen during this process. For example, timestamps and data fields need to be consistent across all applications; otherwise, IT teams risk running into inconsistencies when integrating data that is not all in the same format. These overlooked data entries can leave significant impacts on financial, executive, and managerial teams that rely on accurate data for reporting and decision-making.
An estimated 40 percent of enterprise data is either inaccurate, incomplete, or unavailable, resulting in businesses failing to achieve their data-driven goals. So, a great amount of attention must be directed toward how data is formatted and handled. Otherwise, integrations will include inaccurate data, which is worse than having missing data because those who see it won't know if it's correct or not.
With integration systems, data flow is automated, making the process easier and more accurate. For example, integration tools with staging areas and the publish/subscribe messaging models significantly track, update, and replicate data, all of which support successful integrations and, if necessary, data recovery. MSPs and IT teams can also use these tools to develop data receipts that pinpoint where and when errors occurred during integrations.
Improved communication protocols
Since data integrations support SaaS applications and other applications moving to the cloud, IT teams must understand data structure to avoid formatting and file type errors during the process.
For example, teams need a protocol for how many characters the "describe your problem" textbox can fit within customer ticket records. Otherwise, they risk exporting truncated data or being wasteful with data space. Before implementing an integration software, MSPs and IT professionals need to plan and understand how varying data structures may impact integration results.
Additionally, some notions of data types and display values need to be agreed upon before integrations in order for enterprise customers to conduct meaningful inquiries or even significant data ingestion. From the translation of codes to values, timestamps, and time zones, these sometimes overlook data points can lead to substantial inaccuracies post-integration.
The T-E-L approach
Traditionally, the data integration process follows the E-T-L (extract, transform, and load) approach. However, some integration tools use the T-E-L (transform, extract, and load) method, a more effective way of manipulating data before an integration.
With the T-E-L approach, customers can manipulate their data before copying, extracting, and integrating it, allowing them to control the process precisely the way they want to. Meanwhile, the traditional E-T-L method causes customers to manipulate data in the middle of the process, a practice that is often unpredictable and may not produce the desired results.
The T-E-L method supports a streamlined integration that helps prevent inaccurate data and errors. It also saves time and resources companies would put toward reverting an integration or repeating the process all over again due to inconsistencies.
The future of data integration
Now more than ever, data integration is a critical approach for enabling the capabilities of cloud technology to enhance and grow business goals and operations. With packaged integration software tools, enterprises can easily control and integrate large amounts of data, preventing disastrous outcomes that can be detrimental for business.
Image credit: Tashatuvango / Shutterstock
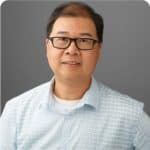
David Loo is a 30-year veteran in systems and applications integration. He founded Perspectium in 2013 and was a founding member of ServiceNow's development team and instrumental in creating the foundation for integrating and extending the platform.