The rise of the analytics engineer

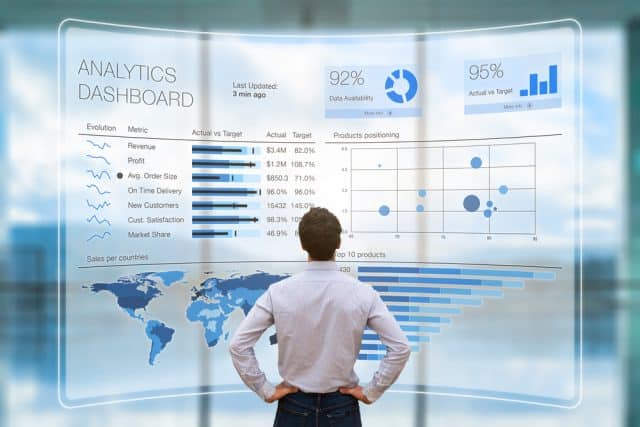
The rise of the analytics engineer pays homage to the evolving complexity of modern data analytics. The role encompasses many fields, and is closer to software engineering than traditional analytics, as it involves writing programing scripts and maintaining data as a set of software-producing artifacts.
It’s within the context of a rising awareness of the data ecosystem that the demand gap between data scientists and analytics engineers is closing. Once heralded as the 'sexiest job of the 21st Century', analytics engineers are set to soon steal this accolade away from their antecedents.
This comes as, for many years, data science and data scientists have been a growth area for organizations digitally transforming and looking to optimise and monetize all aspects of knowledge work and associated data. But despite much investment, the hoped-for operationalization of data models hasn’t panned out as per the high hopes of analytics professionals. Large numbers of data scientists were not able to apply their high skills in a business context. They found themselves dealing with the tedium of messy, disparate data, not the high end of model creation.
But, what are the specific nuances between each of these roles? Do data scientists and analytics engineers employ the same tools and tactics? This article will explore these topics, while offering some predictions and advice for aspiring professionals.
Move Over Data Scientists: Distinct Data Roles Are Converging
Emerging at the intersection of the data analyst and data engineering functions, analytics engineers create data products that include insights and the rigor of backend engineering processes, empowered by the rise of the modern data stack. More technical than 'traditional' analytics translators, they have deep knowledge of SQL. This, coupled with an understanding of the business, is a skill in itself, and it’s been notably absent in many junior data scientists. From this lens, the analytics engineer is perceived to be the missing glue that connects an organization's data to the all-important business context.
And, the role of an analytics engineer is becoming better defined and more efficient, thanks to tools that are designed to work with humans, at cloud scale, including new platforms for defining custom transforms (e.g., dbt), exporting semantic concepts to the business (e.g., metrics layers), and monitoring the results (e.g., data observability systems).
Where the analytics toolset is becoming ever more powerful and accessible, the person controlling this business transformation logic internally is an analytics engineer.
Analytics Engineers Are Using The Best Of Breed Tools
Coincident with the rise of this new role comes a new set of best of breed tools. These have been supporting the changing skill-sets and ambitions of analytics professionals, playing a role in the changing needs and abilities of industry professionals. These tools hail from across the whole cloud-based modern data analytics stack. These are consumer-grade analytics, without which the modern data stack wouldn’t be the useful, usable and democratizing force that it is.
Users are changing the analytics game by incorporating data to and from tools like SaaS
applications such as Salesforce and productivity apps like Slack, Google Docs, and Teams. They are building entirely new data products like data apps that monetize data and opening entirely new revenue streams for businesses. Data science and cloud data apps and platforms have become ubiquitous, and the barrier to entry has dramatically lowered in terms of usability.
The desirable end state is for the analytics professionals, with analytics engineers playing their part, to be creating, consuming, and operationalizing data-driven insights using tools that are simple, open, and actionable. Consumer-grade search and AI technology has come to the fore, delivering true self-service analytics that anyone can use. The cumulative effect is that analytics engineers can sit between analysts at the end of the line -- creating their dashboards or reports, and data scientists -- who want granular, unscrubbed data. Analytics engineers come of age in this world of cloud data platforms and data build tools (dbt).
How To Break Into A Data Role
Analytics engineers first appeared in cloud natives and startups, but recently started gaining ground in enterprise companies, with JetBlue, American Tire, and Guy Carpenter as early adopters. The dbt community started with just five users in 2018. By November 2021, there were now 7,300 users. The demand for data scientists is about 2.6 to 2.7 that of analytics engineers, with the gap closing.
Breaking into the community requires a few skills, all very much in demand, but also a certain attitude and aptitude in the business domain. You might move in from data engineering, but you are an SQL champion, writing and using. Assuming you’re already part of the analytics ecosystem, it’s very possible to deep dive into SQL on the job.
In terms of attitudes, a successful analytics engineer should be very systems oriented, with high levels of comfort managing processes and organizational proficiency. But don’t neglect both the halves that make this role so important. Engineering is one part, the other is business awareness, communication, and partnership. Using business logic alongside software development -- while maintaining a focus on the analysis, is key.
Image credit: NicoElNino / Shutterstock
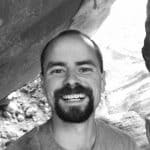
Michael Ritchie is the Vice President of Engineering at ThoughtSpot. Michael founded SeekWell, a pioneer in Reverse ETL, in 2018 which was acquired by ThoughtSpot in 2021. Before founding SeekWell, Michael was the Chief Risk Officer at a Fintech company and led analytics teams at Bank of America and MBNA.