The importance of responsible AI

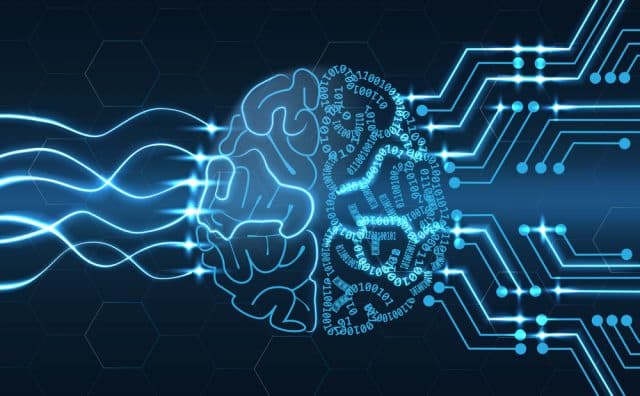
Artificial intelligence (AI) is growing and shows no signs of stopping -- almost. In 2020, IDC estimated global spending on the technology would more than double by 2024 to hit $110 billion. Investors feel the same enthusiasm. CB Insights reported venture capital for AI startups in Q3 2021 reached a record $17.9 billion. Yet, even in the bright light of such success, a shadow is being cast. Even as AI is exploding, trust has leveled out, and that could eventually stall its progress and acceptance if we are not careful.
Given how widely AI is being deployed, many organizations are content to look the other way; so long as there’s value, no need to ask questions. But what about transparency and responsibility? If a company can’t trust its own algorithm, why will consumers? Case in point is the Apple Card launch in 2019 in which a noticeable difference in credit lines offered to men vs. women was revealed. Turns out, a faulty AI design failed to have gender input. Further, Apple hadn’t been following the algorithm closely for bias. That’s how launches and reputations are undermined.
Trust or Bust?
AI is a powerful tool. It drives more effective decisions, adds insight, while streamlining workflows and costs. But you have to be a data scientist to fully understand how it operates and the interactions that occur between inputs and outputs. Most every consumer, and the majority of users, would have great difficulty understanding how results are achieved. You might be able to lower bias, but it’ll never go away, particularly when you don’t know when you don’t know where it exists.
Transparency is particularly elusive when it comes to machine learning (ML) and deep learning (DL) models. The former is often actually often called "black box AI" by data scientists. That’s because ML algorithms use inference and not specific knowledge when seeking details and patterns. ML models must be fed large amounts of data for training and learning, still, explaining conclusions is imperative for customers and stakeholders. Not being able to identify what prompts bias, or flat-out wrong results, is not only irresponsible, it requires you to start again and retrain the system.
DL can be even more difficult to understand. It relies on an artificial neural network that learns without human oversight which enables it to tackle more complex initiatives such as exposing financial fraud. However, on the flipside, it overly saturates an environment with data, so much so it can end causing more confusion that clarification.
Corrective measures can cost a lot of time and money, incur the wrath of the c-level, derail AI trials meant to enlist their support. Still, what’s the alternative? Without that trust, investments can easily be lost.
Responsible, Reliable and Viable
This is not to say we throw ML and DL away, hardly, each has benefits and drawbacks. Plus, there are emerging developments that offer great advancements, symbolic AI being one.
This AI tact has actually been around for a while but is now enjoying a resurgence. That’s because it’s proving particularly useful for natural language understanding (NLU), which can facilitate a platform’s comprehension of normal, everyday speech. And with a rules-based strategy, you can gain complete visibility into the inner works of any model. This means fast detection of flaws in data or an algorithm, which with new rules, can get things back on track. It exposes how AI is being used, making it explainable and more trainable.
Still, all approaches bring something to the table and now companies are able to blend the best elements in a hybrid approach. Remember for success, enterprises must be able to show that they can responsibly and reliably use AI, and the best way to do that is with algorithm transparency. So, to that end, a cocktail of ML and symbolic AI can be particularly potent. The resulting understanding of human language can cull value from unstructured data, which is then supported by the processing horsepower of ML.
Building these types of frameworks are the way to illustrate responsible use of AI and a path to the future. It paves the way for companies to expand business models further and much more quickly. It also allows cost-efficient trial and error, and the ability to course correct without starting from scratch, both of which AI requires.
It only makes sense. Key to the evolution of most successful technology spaces is an ability to combine the best elements. And a hybrid approach is the only way that we’ll be able to construct the frameworks that’ll ensure the responsible, reliable and viable use of AI.
Image credit: Laurent T / Shutterstock
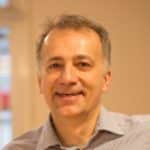
Luca Scagliarini is the Chief Product Officer for expert.ai, where he is responsible for leading the product management function and overseeing the company’s product strategy. Previously, Luca held the roles of EVP Strategy & Business Development and CMO at expert.ai and served as CEO and co-founder of semantic advertising spinoff ADmantX. He received an MBA from Santa Clara University and a degree in Engineering from the Polytechnic University of Milan, Italy. Luca blogs regularly about the real-world applications of AI and cognitive computing for today and tomorrow.