How web scraping has gone from niche to mainstream [Q&A]

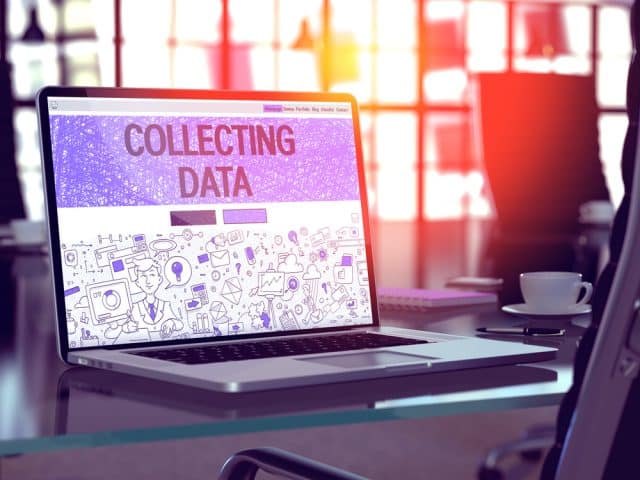
Web scraping -- collecting data from websites -- has been around almost as long as the internet has existed. But recently it's gone from a little-known niche to a serious activity, using automation to collect large amounts of information.
We spoke to Julius Černiauskas, CEO of data acquisition company Oxylabs to find out more about web scraping and how it has evolved.
BN: How has web scraping technology been changing over the years? Have there been any significant developments?
JC: The development of web scraping is intertwined with the development of the internet. As websites are becoming more advanced, web scraping needs to keep up and advance too in order to remain effective. Hence, a lot of recent web scraping developments are about meeting the complexity of modern websites, adapting to constant changes and remaining flexible.
Another drive for the technology's development has been the growing diversity of its users. Web scraping has long been seen as a niche technology mainly used for several specific use cases. However, the use of technology becoming heavier and more industries discovering it has fostered new technological advancements. The feedback from the clients helped the developers of web scraping solutions identify previously unknown challenges and set out to solve them.
As a result, web scraping tools are getting more specialized. A good example of such a transition is our own family of Scraper APIs -- a trio of different products, all dedicated to a specific use case. SEO companies want tools that deal specifically with SERP scraping challenges. Ecommerce companies, meanwhile, focus on different features. Web scraping customers want dedicated, often even personalized solutions.
BN: What have been the main innovations in web scraping in recent years?
JC: Artificial intelligence and machine learning are game-changers in web scraping. With their help, common web scraping challenges can be solved before the user even starts noticing them.
There are several ways in which AI and ML benefit web scraping. For example, a few years ago we launched Next-Gen Residential Proxies. There, AI powers dynamic fingerprinting -- it imitates an organic user's behavior, meaning that the customer can collect data undetected and without getting blocked.
Another feature enabled by AI and ML is adaptive parsing. It is especially relevant to eCommerce companies, who want to collect the same type of information from different websites, for example -- product title, discount, price, etc. While different websites have different layouts and even tend to change them once in a while, AI-powered adaptive parsers detect the needed information without hassle.
Such and similar technology enrichments with AI and ML make web scraping easier and more efficient, as there's no need to spend time solving common challenges such as getting blocked, solving captchas or collecting the wrong data.
BN: There have been many conversations around data and its privacy in recent years. Has this affected the web scraping industry and its perception?
JC: It's a sign of progress that people are starting to take personal data and its privacy seriously. Personal data could be a sensitive issue and must be treated carefully. Many people now understand it and are more actively participating in decisions that concern their own personal data. For example, some tend to read various terms and conditions more carefully and do not necessarily consent with them all, some even consider if they really need an app that collects that much of their data -- it's becoming an increasingly important topic.
However, while consumer knowledge about personal data is growing, the whole concept of public data is less discovered. For this reason, web scraping as a technology is still often misinterpreted and misunderstood – you need to understand the concept of external data first to understand the technology that helps to collect it -- web scraping.
Other misconceptions that remain are that web scraping is a very complicated technology or that it can only be used by the largest corporations. In fact, it is becoming much more accessible and simpler to use.
BN: Have you seen any changes in demand for web scraping recently? Who is the typical user of the technology?
JC: The demand has been growing exponentially for the past few years. The global pandemic was a major catalyst -- businesses were suddenly moving online and the competition in the digital world got enormous. To get a chance in this extremely competitive environment, many businesses started strengthening their data departments. Furthermore, even those companies that haven't collected external data before, started experimenting with it. The use of web scraping tools grew massively.
The typical user of web scraping is a company that understands the value of data. Usually these are businesses that operate in a global or a very competitive market and thus need to get original business insights that would get them ahead in the competition.
BN: Where is this technology used the most?
JC: There are very specific types of businesses that base their whole model on web scraping. For example, price comparison websites, flight fare or hotel fare aggregators critically depend on web scraping everyday. Without this technology, such business models wouldn't even be possible, making it harder for regular consumers to compare options.
The eCommerce industry is one of those where web scraping is indispensable. Ecommerce companies depend on external data collection for market research and competitor analysis -- for them getting to know the competitive landscape is crucial. Price intelligence is another one of the most popular uses of web scraping. Most online stores use it to adapt their own pricing, decide on discounts or implement dynamic pricing.
Finance companies are also heavy users of web scraping. In fact, our recent research revealed that 71 percent of finance companies in the US and UK use web scraping for their decision making. Some of the most common uses involve market research, evaluating companies and discovering data-backed investment opportunities, and risk management.
BN: What should businesses consider before starting to collect external data?
JC: First of all, consider what kind of data and from what websites you plan to collect. Make sure that the data you want to collect is public and doesn't involve any personal information.
Be aware of legalities around the data you plan to collect. It's always a good idea to have a legal professional around to evaluate your data collection plans and make sure you only opt for best practices for gathering data.
Secondly, consider which way of managing web scraping infrastructure works better for your case. Are you capable of dedicating a team and managing it internally, do you want to completely outsource web scraping solutions, or would it be better to find a middle ground between those two options?
Large corporations usually build their own infrastructure and have dedicated teams to support it, so usually they only need to buy proxies in the market. For smaller companies it might be easier to outsource ready-made web scraping solutions, so that they can focus on analyzing the data instead of the challenges of collecting it.
BN: Can you offer any practical tips for companies that collect public web data? How can they make the process more efficient?
JC: I still often see companies that focus on collecting a lot of data without even considering if they really need it. However, data has as much value as the insights you can draw from it. Therefore, start by clearly defining the aims of the data you plan to collect. And remember, it's better to collect less data, but rather focus on its quality.
Another tip that I could give is don't be afraid to try out new solutions. The technology is developing so fast and constant upgrades are being delivered. We ourselves are constantly innovating and introducing new products and features that make the process even more efficient. As I've mentioned, AI, ML and other advancements are changing the way we collect data so that we can focus on a more important part -- analyzing it.
Image credit: Tashatuvango / Shutterstock