Marketing data science -- what is it and where is it headed? [Q&A]

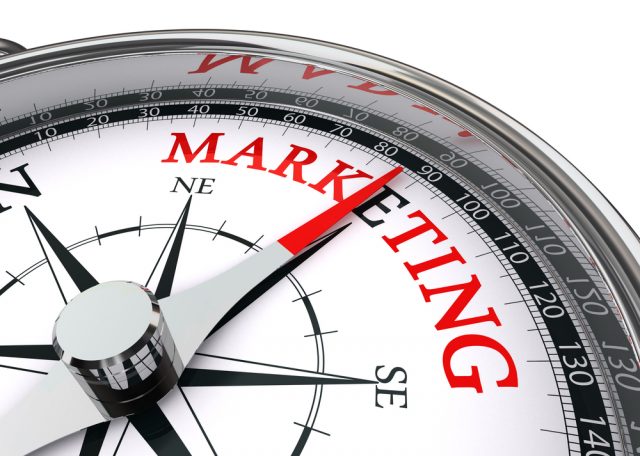
Data science involves analyzing and interpreting large, complex amounts of data and turning it into valuable insight to achieve business goals. Though not exactly a breakthrough field now, it has changed many aspects of how markets conduct business.
In a conversation with Jerry Johnson, founder and president of Marketing Data Science Associates and director of Cascade Strategies, we talk about data science, its applications in marketing, and its future with the emergence of AI.
BN: What are the most common applications of data science in marketing?
JJ: Several data science methods are currently being used by marketers all over the world. Nowadays, most companies are collecting vast amounts of information about their customers and storing them in their various databases, so that they can optimize their marketing efforts. One of the most common ways in which data science gets applied in marketing is through recommendation systems, which lets users select from a menu of recommended items customized according to the specific user's browsing history.
Then there's also Churn Prediction, which is a machine learning technology that can tell marketers the likelihood a specific user will leave their site, cancel an order or unsubscribe from their mailing list. It's a powerful tool for customer retention. Other than some sophisticated machine learning and AI tricks, the reason data science is becoming such a favorite among marketers across the globe is because of how powerfully it can optimize traditional marketing strategies such as segmentation, targeting and positioning. data science can make all three of these extremely personalized and effective for companies who know how to leverage the wealth of data.
BN: Has data science changed marketing from being an art to a science?
JJ: I wouldn't say that it has changed marketing to a scientific process completely, as marketing still has many aspects that require human creativity. Data analytics can give you accurate ad projections, sure, but someone still needs to write the copy. data science, at least till now, has not shifted marketing from the creative domain to a scientific one, at least not completely. A marketing data scientist can find answers to questions such as 'Who are your potential customers?' and 'How do people feel about your brand (sentiment analysis)', but at the end of the day it is still the creative team who will have to implement out-of-the-box methods of targeting the stated customers and responding to popular sentiment and brand perceptions. So from this example, we can see that what data science has done instead is that it has made the creativity of marketing teams much more effective and backed with reasoning. We are not shooting arrows in the dark anymore as we now have data that is much more reliable and predictable than before. In other words, Marketing data science has streamlined creativity.
BN: What's the difference between marketing data science and marketing analytics?
JJ: They have plenty of things in common, such as data visualization, data queries, and deriving data-driven insight, but both still have several key differences. Analytical tools are used by data analysts to interpret and analyze historical marketing data. Analytics, a branch of data science, is effective at spotting historical trends and conveying the outcomes of prior performance. On the other hand, Marketing data science is effective at comprehending how all first-, second-, and third-party data may be used to obtain competitive business information. Marketing data scientists are essentially business analysts that focus more on predicting the effects of upcoming actions than reviewing past patterns.
While data science is required to respond to more challenging issues about the future of the company, analytics is required to comprehend previous marketing effectiveness. To extract marketing intelligence from data and transform that intelligence into business knowledge, you need both disciplines and tools.
BN: Why has including data science in the marketing mix become so important?
JJ: Data science in recent years has become an indispensable part of the marketing mix. Many companies nowadays include data science from the early stages of the marketing funnel itself for higher returns on investment. Basing your secondary strategies on data-backed primary marketing funnel strategies helps brands make better decisions, predict trends and future opportunities, optimize budgets, refine audience segmentation and targeting, accurately examine brand perception, improve customer experience and plan a more precise and relevant content strategy.
Businesses can't truly leave data science out of their marketing plan in a world when more than 2.5 million gigabytes of data are created every day. If they do, it won't just be expensive for them; it will also impede their progress.
BN: Do you believe that AI will transform data science? How will it impact the world of marketing?
JJ: Artificial Intelligence is essentially the next step in data science. In a time when businesses are continuously looking for new methods to sell to an oversaturated audience, AI and Machine Learning are greatly enhancing the efficiency of the data science process and providing us with previously unheard-of access to customer insights.
With the help of AI, we are going to have more sophisticated predictive analytics and data tiering -- an automated prioritization system that shifts heaps of cold (inapplicable or less- utilized) data to less expensive long-term storage for access and retrieval when necessary, and freeing up more valuable space for more useful, active, and dynamic data without requiring someone to perform this separation manually. With the growth of AI technology, cloud-native analytics will become much more vital to the marketing process and the data-as-a-service [DaaS] model will rise in popularity.
This is not to say that AI robots will replace data scientists in the industry. We know that no matter how advanced the AI technology may be, it will still need human intervention for handling complex or extremely new data. Besides, machines don't have soft skills or intuition. At least not yet.
Image Credit: donskarpo / Shutterstock