Storage challenges in a world of high-volume, unstructured data [Q&A]

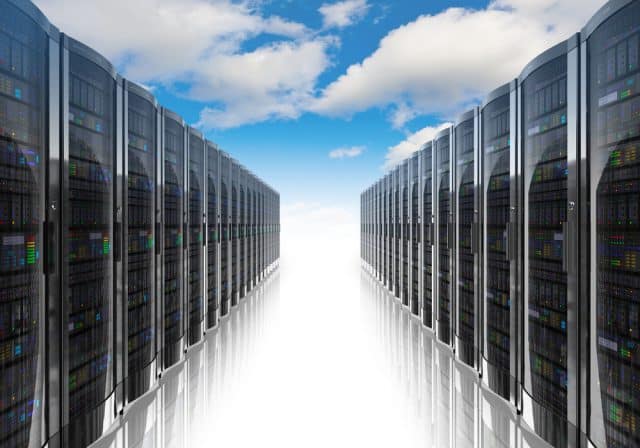
The amount of data held by enterprises is growing at an alarming rate, yet it's often still being stored on 20-year-old technology.
Add to this a proliferation of different types of systems -- or even different storage platforms for specific use cases -- and you have greater complexity at a time when it’s hard to find new IT personnel.
We spoke to Tim Sherbak, enterprise products and solutions marketing at Quantum, to find out how data storage needs have evolved over the last decade and how organizations can cope with the explosive growth in data-rich technological advancements, including virtual reality, AI, and machine learning.
BN: Why hasn't storage kept pace with the growth in data quantity?
TS: I think it has been keeping pace -- until now. Most enterprises still use disk-based storage, which accounts for about 80 percent of the market, and these systems have scaled to meet past growth. At the moment, if you need more file storage, you can simply buy more disks. But the difference now is that the type of data being created has changed to unstructured, and consequently it is much larger than ever before. Plus, it is being kept and reused for far longer.
Prime examples are applications using AI and ML which need storage capacity on a completely different 'hyper' scale. Additionally, there are increasingly lengthy compliance requirements for data retention. Also, other functions are asking for additional storage, like marketing departments. They are retaining customer intelligence for longer so that it can be enriched and reused, and want to keep their unstructured content such as videos, podcasts, etc.
The projected growth of unstructured data is phenomenal, with Gartner predicting that it will triple in just three years from 2023. With this in mind, customers are realizing that their existing disk-based solutions are reaching the end of their road map and will no longer be able to meet their storage needs in terms of cost, capacity, and performance. So, they are starting to look for alternatives to their legacy systems. Ready to take their place are modern, innovative technologies that provide high-performance, cloud-native solutions for unstructured data and file storage with massive scale-out architectures designed for flash and RDMA networking.
Now is the right time to take stock of the research such as IDC's and plan ahead for exponential growth.
BN: Why is unstructured data a particular issue?
TS: It's an issue because unstructured data is far larger than traditional data sets and requires huge amounts of capacity. But it's no longer just a storage size problem. Increasingly unstructured data is being retained and re-used for decades which means accessibility and performance are much more important factors than they used to be. More of this intelligence is now regarded as hot data that can't be consigned to slow, poor performing storage. Users need to be able to find relevant data quickly so it has to be searchable too. Therefore, it must be properly categorized, have assigned metadata, and be easily transferable. It also needs to be cleaned up by removing duplicate data. Many enterprises have information spread across multiple systems, or some in the cloud and some on-premises. And they don't know exactly what they have or if they are holding copies of the same data in numerous places.
Organizations clearly recognize the untapped potential in the data and intelligence that they are storing, but they are struggling to harness it effectively to unlock its true value. Modern storage technology will bring new levels of automation, performance, and flexibility to this unstructured data without the old constraints of outdated hardware.
BN: What is 'hyperscale' data and why is it different from big data?
TS: Hyperscale is the next evolution of big data. We are going to see companies that were dealing with millions of files of data in petabytes move into the league of hyperscale as they manage billions, even trillions of files and objects, amounting to exabytes. Its main difference to big data is the sheer scale of it as its name implies, but it is also about having the foresight to plan how that mass of data will be controlled and utilized.
Current hyperscalers are the likes of AWS, Google, Apple, Facebook, Azure, who have built their organizations on the acquisition and clever management of enormous amounts of data. Now, through their pioneering use of ML and AI they are also creating vast tracts of new data which they need to store, reuse, and manage securely. These first hyperscalers have driven new requirements for storage solutions that enable them not just to store greater volumes of data but to be able to use and manipulate that stored data much more effectively.
As other large enterprises start to leverage ML and AI they too will face massive growth in their storage requirements, along with the challenges of how to access and derive maximum benefit from it.
BN: What security and compliance challenges do the extra volumes of data present?
TS: Ensuring security across the lifecycle of data is already a challenge for organizations and will become exponentially harder as volumes grow and the internal movement of data increases.
Modern storage solutions can proactively manage data to optimize performance. This means that data will reside in different places within the infrastructure while it is processed. It may move into different cloud platforms and then be retained in an appropriate edge location. Maintaining cyber security and resilience across the entire lifecycle of this much more fluid data will need to be increasingly robust as attacks from criminals will continue to become more sophisticated.
Another challenge, for both compliance and security, is the ability to query large volumes of data quickly and easily. Responding to compliance regulations and requests with legacy systems can take days to retrieve the relevant data. Similarly, if companies are trying to identify vulnerabilities or contain a malicious attack they need to be able to quickly search data to detect and fix issues. This requires an architecture that can query information instantaneously -- potentially millions of files across multiple sites -- which cannot be done at speed with disk-based systems. As data volumes accelerate, security and compliance will be key drivers for modernizing infrastructures.
Storage providers are also becoming proactive about cybersecurity and it won't be long before detection of ransomware is included in their solutions.
BN: Do we need to review policies and practices around data retention?
TS: The reasons for keeping data are evolving, which makes it an ideal time to review policies and practices. More often than not, organizations have treated their data archives as a liability. They are storing them according to legal and compliance regulations, and deleting them as soon as that obligation expires. Now, many businesses want to utilize all kinds of data for extended periods of time to find new ways of monetizing it and extracting further value.
Take an example like ChatGPT that required a giant data set to train it initially. That data needs to be retained securely so it can be re-trained and enhanced in the future. As a result, long-term retention policies will need to be put in place to manage and protect such critical information for many years to come.
Infrastructure leaders should think of their data as a valuable asset, instead of a liability, and ensure that going forward this way of thinking is built into their policies for data management, retention, and security.
Image credit: Oleksiy Mark / Shutterstock