The top five capabilities SIEMs should have for accurate threat detection [Q&A]

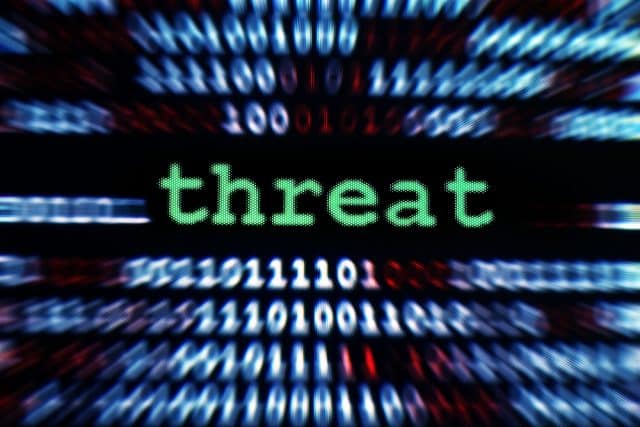
Security Information and Event Management (SIEM) platforms are the centerpiece of many organization's security controls, but if these products aren't configured correctly they will produce too many false positives to be useful, and can even make overall threat detection worse.
Security analysts need to trust that their SIEM is detecting threats accurately. We spoke to Sanjay Raja from security analytics company Gurucul to discuss how SIEMs can be configured to offer accurate detection.
BN: What are the most important security trends affecting SOC teams right now? What SIEM features or capabilities help respond to or manage these trends?
SR: Overall, the Security Operations Center wants to maintain accurate threat detection as their networks become more distributed, complex and increasingly located in the cloud. This breaks down into five specific trends:
- Current SOC solutions struggle to adapt to complex enterprise deployments that require support for distributed, hybrid and multi-cloud architectures. Native support for these architectures is becoming very important for SIEM products.
- SOC teams need to ingest more data sources and support data ingestion seamlessly across the enterprise to get better visibility as they migrate to the cloud. If they cannot access or analyze data from the cloud, those parts of the network become a black box where threats can hide undetected.
- Controlling licensing costs is a big trend in the SOC as data volume increases overall (the cloud is a significant new source of data being generated). Pricing models based on the amount of data ingested are becoming less popular because they penalize organization for better visibility and more accurate detection.
- More data sources and a higher volume of data being ingested also creates a flood of events and false positives. Security teams are looking for solutions that help them filter the noise despite this increase in alerts to sift through.
- SIEMs have always been challenged to accurately detect individual threats while also gathering the right context to understand the full attack campaign. Security teams are looking for a wider breath of attack detection models to detect a wider variety of known threats and variants, but also gather the needed context to investigate and validate entire attack campaigns.
BN: False positives and floods of information from external threats are a challenge for many organizations. What are three capabilities that SIEMs should possess for accurate threat detection to combat these threats?
SR: First, the ability to easily ingest data from new applications, systems or devices. Gathering more data is important, but the ability to automatically parse that data without customized parsers provides immediate visibility when supporting new or updated applications, systems and devices. This allows for more security relevant telemetry to monitored actively while ignoring non-security related events and context.
Second, threat detection based on advanced, trained machine learning. Rule-based ML are essentially a static flow chart that is only useful as long as a threat model with these rules has been built to find a specific attack. SOC teams need trained ML that can better adapt to the organization and trigger based on the data it sees and monitors. This lowers the number of false positives that can be incorrectly triggered, and with the non-fixed and larger set of unrestricted telemetry can better identify new variants or unknown attacks.
And third, link-chain analysis, which is the ability to chain multiple threat models and analyze them together. For example, being able to understand how unusual behavior associated with a large file transfer and/or dangerous network activity can be pieced together as a high-risk security event. Security teams looking to reduce manual efforts in security operations can leverage this capability to lower overall detection and investigation time as necessary context becomes readily available as well.
BN: What are the implications for the SOC as organizations continue to migrate servers, data, and other sensitive information to the cloud? How does cloud use affect SIEMs?
SR: Security teams are often surprised at the sudden increase in logs and event data generated by cloud infrastructure versus on-premise systems and applications. In addition, most SIEMs have trouble gathering data and searching across multi-cloud and distributed cloud environments, for example, data stored across two different AWS regions. Another problem is the reliability of cloud data. Security analysts are asked to be experts in cloud configurations across AWS, Azure, GCP and other providers, yet each one is different and changes rapidly. This is no small task to be familiar with the architecture of all important cloud providers to make sure the SIEM is leveraging all the necessary data.
BN: If an organization's SIEM is lacking some of these capabilities, what are their options? Should the SIEM be used alongside other threat detection platforms? What are the advantages and disadvantages of leveraging multiple platforms?
SR: The rise of XDR (extended detection and response) started because the SIEM lacked many of the capabilities explained above. However, as XDR has simply become an extension of EDR (endpoint detection and response) in many cases, it continues to lack the parsers, telemetry and analytics to effectively detect threats. It has not lived up to the hype in terms of efficiently detecting threats, automating manual processes, and ultimately preventing a successful breach. So, it has become mostly a data aggregator across security telemetry with siloed analytics. As mentioned above, most traditional SIEMs lack cloud support.
This means some organizations have been using a cloud specific SIEM alongside their traditional SIEM. This provides the necessary visibility, but is very costly to buy, maintain and staff while the organization still maintains their legacy SIEM. What we will begin to see is next-generation platforms that are cloud-native, support complex architectures and incorporate XDR features while making proper use of analytics and machine learning to build faster responses.
Image credit: alexskopje/depositphotos.com