Five tips to inform your AI and data science strategy and maximize your investments

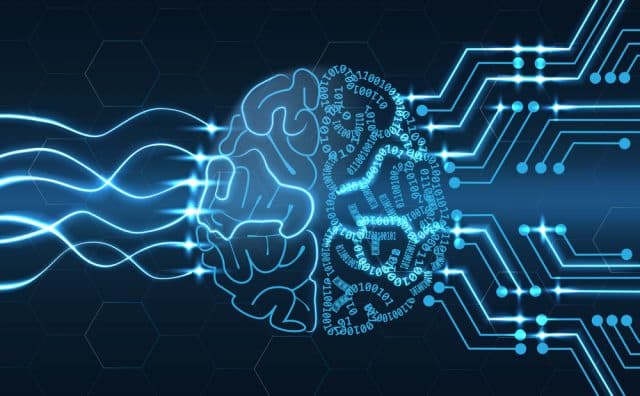
Data science and AI leaders are rushing to accelerate new technology adoption. According to Forrester, generative AI alone will see an average annual growth rate of 36 percent for the next seven years, taking 55 percent of the AI software market. The analyst firm also estimates that by 2030, $79 billion will be spent annually on specialized generative AI applications and $42 billion will be spent annually on generalized generative AI use cases.
Even if Forrester is off by a few billion dollars, moving fast is still critical to achieving success, but so is having an optimal AI strategy. Leaders must ensure that practitioners have the technology they need to innovate. At the same time, they must ensure innovation does not exceed budgets or introduce new risks.
For more than a decade, companies have invested heavily in data operations. Now, they look to enable their data science teams to extract insights from the data. Leaders quickly discover that to maximize the return on their investments, they must balance their expectations against budgetary discipline. The solution lies in defining the optimal data science strategy.
AI's potential is consequential. A recent study found that nearly all (98 percent) CDOs and CDAOs say that companies that bring AI products to market fastest will survive and thrive in times of economic uncertainty. Nearly all (95 percent) say these exact solutions will enable them to achieve a revenue boost.
However, we consistently hear from AI leaders that many of their projects need to overcome broad barriers and end up wasting money. Worse, these leaders say their models fail to scale as a result of inadequate infrastructure investments. There are no magic bullets, but we've seen the world’s most sophisticated companies benefit from incorporating these five cost-saving tactics into their AI strategy.
1) Defeat Data Science Silos
Data science silos are a fact of life in all large organizations. To use their data, business units organically build data science teams, leading to duplication of efforts that waste resources. This ends up also stifling knowledge sharing, agility, and innovation.
In contrast, a holistic approach enables different teams to collaborate. The right tools simplify collaboration for groups located on the same floor or distributed across global regions. This focus on maximizing the value of resources, practices, and outputs will power the organization to achieve a competitive advantage through AI.
Keeping the "3 Rs" in mind -- reduce, reuse, and recycle -- is essential. Companies accelerate innovation by building on prior projects and experiments. Instituting the 3 Rs across the organization helps tear down silos. Reuse makes it easier and faster for teams and individuals to be productive more quickly, reducing if not eliminating mundane configuration tasks.
Giving teams access to existing code and models enables the data science practice to grow organically across business units. Each can choose what works best for them and build on what worked best for others. The outcome: your organization increases innovation while cutting time to value and costs.
2) Don't Let Models Die on the Vine
Data models that don't get deployed quickly (or at all) waste time and money, and allow the competition to get ahead. To enable and accelerate model deployment, enterprises must look for opportunities to automate and reduce manual effort. Automating infrastructure provisioning lets data scientists overcome deployment barriers. Instead of relying on DevOps or IT, they can roll out a model as a dashboard app or an API. It also spares them from becoming rogue IT operators. Naturally, faster model deployment drives value and increases ROI.
Models in production require constant monitoring. Automated model and data monitoring can ensure they remain relevant. The bottom line: the sooner your models are validated, the sooner they can scale and contribute to ROI and AI success.
3) Think Outside the Cloud
Cloud costs increase once a business begins to scale its AI operations. Data science demand, however, fluctuates wildly. You should adopt a hybrid- and multi-cloud strategy to meet this demand. Mix and match your data center capacity with the best the cloud offers. Even if you are sticking with the cloud, be smart about using the right tools for the job. Use high-end GPUs to accelerate model training and deep learning inference. But choose lower-cost CPUs or older GPUs for statistical analysis or data cleansing.
Data transfers are another line item to watch for in your cloud bill. Model code is relatively small. As a result, you can save money by moving and running models where the data lives instead of moving the data around. This approach cuts costs while improving data and privacy regulation compliance. Another point to consider: In some situations, you can save even more money if you buy GPUs for your data center. Owning often ends up cheaper than renting GPUs in the cloud.
4) A Productive Team Is a Happy Team
To increase efficiency, start by identifying productivity barriers. For example, data scientists waste time waiting for infrastructure resources and data. They waste time recreating assets and configuring development environments. They waste even more time getting trained models into production by themselves. Besides the financial impact, all that waste and waiting can have an expensive side-effect: Data scientists are in high demand. Retaining them will be difficult if they get an offer from a company they feel values their skills and doesn't waste their time.
Statistics suggest low productivity's impact on building a winning data science team.
- 20 percent of data scientists switched jobs over the last 12 months; of those, 51 percent saw salary increases of more than 20 percent. Money is a factor, but so is removing roadblocks and allowing them to grow their skills.
- 78 percent of organizations find hiring data scientists difficult, with more than half of organizations focusing merely on backfilling roles.
- 99 percent of chief data officers felt their organization's choice of tools impacts the hiring and retention of data scientists.
These stats help explain why automation will not just make your data science teams more productive. Automation and better tooling make hiring and retaining high-quality data scientists easier.
5) Practice an Ounce of Prevention
Irresponsible AI and lack of governance can lead to significant risk: reputational damage, bad press, regulatory fines, and lost revenue. According to The National Law Review, "2023 is shaping up to be the year of U.S. privacy laws!" Companies must catch up on numerous new federal and individual state rules and new regulations from Europe. Violations, even for unavoidable data breaches, can bring hefty penalties.
Also, remember the lessons learned from companies that failed to account for model risk. Why? Reputations take decades to build. A wrong result from an outdated model can impact customers' lives. Beyond regulatory fines, customers and prospects will stay away from your products if they see you mishandling their data or using it against social norms.
Responsible AI is the only AI you should practice. It requires proper governance with end-to-end tracking, monitoring, auditability, and reproducibility. There is no "set it and forget it" in data science. Build a strategy that ensures your team's data, code, libraries, and frameworks can be accessed and validated. Internal and external auditors will need to know how you made each decision and prediction. Without the right tools, the process can be painful or plain impossible.
Your Strategy Is Your Roadmap
You need an optimal AI strategy that makes innovating easier and eliminates waste. It will encompass concrete cost savings, increased infrastructure utilization, and better productivity. It also empowers teams to adopt responsible practices. Best of all, you get to keep your job. The five areas outlined above provide a starting point. While no two companies are alike, you can adapt your practices to your organization's needs. Maximizing the return on AI investment will not only help your company take a market lead, it will also demonstrate why you deserve your next promotion and raise.
Image credit: Laurent T / Shutterstock
Yuval Zukerman is Senior Director, Domino Data Lab.