How to build a successful data lakehouse strategy [Q&A]

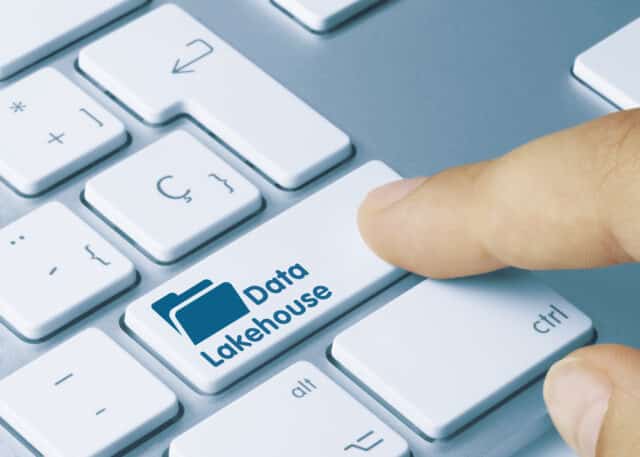
The data lakehouse has captured the imagination of modern enterprises looking to streamline their architectures, reduce cost and assist in the governance of self-service analytics.
From data mesh support to providing a unified access layer for analytics and data modernisation for the hybrid cloud, it offers plenty of business cases, but many organizations are unsure where to start building one.
We spoke to Jonny Dixon, Senior Product Manager at open data lakehouse platform Dremio, to discuss their benefits and how to make the most of them.
BN: Where did the concept of the data lakehouse originate from?
JD: Data is the compass that guides decision-making, helping leaders navigate choppy waters with confidence. However, in reality, data is siloed and stored across a business -- and myriad sources -- in multiple clouds and on-premises. But when a company can't put their data into the hands of those who need it quickly enough, they fail to realise its value and make critical decisions without a complete picture.
However, breaking down silos is expensive, time consuming and risky. The most common work-around for these challenges is making data copies. But this degrades data quality and reliability -- there's no longer a single source of truth -- and data is often stale as it takes time to integrate it from the data lake into analytics and BI tools. Further, in complex regulatory environments, leaders worry they'll lose control of their data if it's shared, copied or duplicated across platforms and the business. Securing and governing data at scale is a complex task given its manual, error-prone and often applied inconsistently, exposing businesses to compliance risk.
The data lakehouse is designed to solve all these issues. It brings together the structure and performance of a data warehouse with the flexibility of a data lake, allowing for high-speed data transformation and querying, and the consolidation of multi-structured data in flexible object stores. While still early on in its adoption, many businesses see data lakehouses as an efficient way to cut costs, facilitate the governance of self-service analytics and streamline their architectures.
BN: What are the benefits of the data lakehouse?
JD: There are two primary advantages of the data lakehouse. The first is simplified architecture. The ability to perform super-fast queries directly on object storage eliminates the need to copy or move data to meet business intelligence performance requirements. This minimises the reliance on data warehouses and data extracts, which in turn reduces the time and effort involved in managing multiple copies of data, and overall costs.
The second major benefit of the data lakehouse is workload consolidation. The data lakehouse is able to support both data science and business intelligence workloads, removing the need for organizations to have separate platforms and streamlining workloads. Those lakehouse platforms that consolidate data views into a semantic layer further enable self-service capabilities, governance and simplify data access. With the data lakehouse, data scientists and analysts can prototype new approaches without moving or duplicating data. The huge benefit of this is that it reduces data engineers' workload, so they can spend their time on more business critical tasks.
BN: How can you execute the right strategy to implement a data lakehouse?
JD: While the benefits are clear, many organizations don't know where to begin with implementing a data lakehouse. In order to make the most of data lakehouses’ potential, enterprises need a modernization strategy and roadmap, starting with an honest assessment of where they stand today. The next step must be to define and prioritize the most essential business use cases for the data lakehouse. Whether artificial intelligence / machine learning projects or interactive dashboards and periodic reporting, the use cases that can deliver a 'quick win' should be pushed to the top of the pile. From there, the architectural characteristics required to support them -- simplicity, accessibility, high performance, unification, cost-effectiveness, openness or governance -- should be prioritised.
It's essential to assemble the right team of people to succeed, including a board-level sponsor, data analyst or scientist, architect, data engineer and governance manager. Together, this 'A Team' can create and implement the plan of action for step-by-step changes to their environment. In practice, this could mean migrating semi-structured customer data from HDFS to a cloud object store in the target lakehouse if the aim is to support 360-customer views. Once the first project has delivered tangible business value, the core team should be able to unlock the budget, architectural platform and senior stakeholder support required to start new projects.
Image credit: Momius/depositphotos.com