What's the best option for businesses -- open-source or commercial AI services?

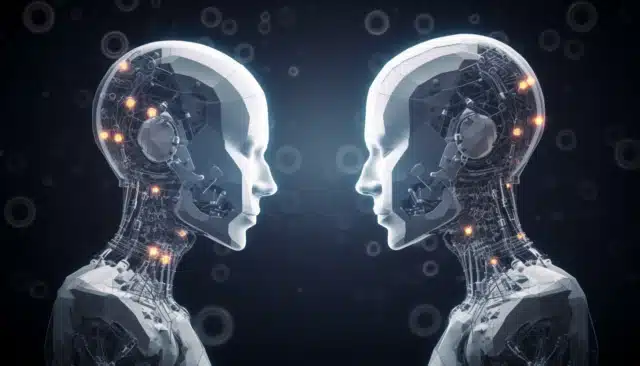
In the past year, Generative AI (GenAI) availability for businesses has swept the market, offering significant boosts in productivity. To successfully seize this opportunity, however, businesses will need to ensure they invest in the right solutions.
Faced with options like commercial AI services and customizable open-source Large Language Models (LLMs), business leaders must navigate a complex landscape of risks and benefits. This choice, influenced by factors like speed to market and data security, is crucial for companies looking to strategically invest in GenAI.
Commercial vs. Open-Source AI: Weighing the Options
Commercial GenAI services, known for their ease of integration, offer businesses a straightforward way to adopt AI. These platforms are designed for immediate use, eliminating the need for extensive setup or resource allocation. They come “enterprise ready” with robust security features and are often in line with data regulations. However, there are concerns about how these services handle sensitive data, with the risk of proprietary information being used in their training sets. Additionally, limitations in content filtering and the possibility of less accurate AI responses pose challenges. For example, a study on ChatGPT showed it could correctly answer 16 out of 21 questions, but its responses tended to be more cautious than a human's.
In contrast, open-source LLMs like Mistral, BLOOM and GPT-J offer a unique advantage -- customization. They allow businesses to tailor AI models to their specific requirements, leading to more precise and relevant results. These models enable businesses to develop their AI tools for various tasks, offering enhanced security through customizable controls. Because of this, companies must be cautious during their data input to avoid training biases in the AI. Overall, LLMs require more in terms of operational investment and specialized skills for effective management, so companies must consider their budget and resources when thinking about implementing LLMs.
The choice between these two options hinges on several factors including data privacy, costs, and the level of control a business wishes to exert over its AI systems. This decision-making becomes more pressing in light of a recent McKinsey survey, which reveals that 40 percent of top executives anticipate increased investment in GenAI by 2024. This trend underscores the urgency for businesses to strategically assess their AI investments, ensuring they align with their data privacy, cost, and control preferences.
Understanding the Security Risks
Commercial AI services, such as GenAI systems, offer a robust security framework, yet they don't automatically ensure the protection of sensitive conversation data. In addition to safeguarding intellectual property, businesses must be vigilant against sophisticated cyber threats that exploit AI technologies. Enterprises must prepare for the possibility of malicious actors using GenAI systems for cyberattacks and fraud. A particularly concerning tactic is 'prompt injection,' where hackers manipulate AI, like ChatGPT, to divulge sensitive information. It’s crucial for companies to consult with their cyber insurance providers to verify the extent to which AI-related breaches are covered in their current policies. Implementing comprehensive security measures and understanding insurance coverage are pivotal steps in safeguarding against AI-exploited vulnerabilities.
Conversely, open-source AI models that lack built-in comprehensive security require companies to create their own robust defenses, including measures against prompt injection attacks, and tailored policies for access and authentication.
Legal and Regulatory Considerations
When selecting an AI solution, legal and regulatory compliance is crucial. Commercial AI services must adhere to international data laws, such as Microsoft's compliance with EU data residency laws requiring EU citizen data to be stored within the EU. This is vital for businesses with European ties. Additionally, using these services involves understanding contractual details regarding liability for data breaches and handling AI-generated content. Privacy concerns and data leakage risks are notable, highlighted by instances like legal actions against OpenAI for alleged unauthorized use of content in training its models.
Open-source AI, on the other hand, shifts regulatory compliance responsibility to the user. This includes adhering to copyright laws and staying updated on varying global AI regulations. Businesses employing these solutions must be vigilant and regularly consult legal experts to navigate the changing legal landscape of AI, especially when operating internationally, to ensure continual compliance and minimize legal risks.
Guidance for a Balanced Approach
Navigating the complexities of these options requires strategic decision-making that aligns with a business's operational requirements, security framework and compliance obligations. Open-source LLMs offer extensive control and customization but demand substantial investment in internal infrastructure and skilled personnel for security and management. Conversely, commercial AI solutions like Microsoft Azure OpenAI Service are recommended if a business is looking to focus on security and regulatory compliance. However, these should be supplemented with additional in-house controls, including tailored content filtering and accuracy management strategies.
Crucially, implementing either solution necessitates integrating content filtering systems that align with enterprise policies and legal requirements to ensure compliance and manage potential risks. Based on data classification and role-based access, layered security protocols are essential to tailor security measures to the chosen AI solution.
Organizations planning to integrate AI into their workflows must keep up with the legal and regulatory landscape. Continuous engagement with legal professionals for up-to-date compliance and risk mitigation is vital, especially in a landscape characterized by rapid technological advancements and changing regulations attempting to keep up.
As organizations increasingly adopt Generative AI, choosing between commercial and open-source models is the challenge. Organizations must carefully assess their priorities when selecting AI solutions. Beyond the imperative considerations of security and legal compliance, it's crucial to evaluate the accuracy, customizability, and cost-effectiveness of these technologies. Aligning AI choices with operational goals involves a balanced consideration of these key factors, ensuring that the selected systems not only meet regulatory standards but also align with the specific needs and budgetary constraints of the business. Navigating this terrain demands a strategic approach that best fits the needs of each individual business.
Dr. John Prichard is Chief Product Officer at Radiant Logic.