The double-edged sword: Navigating data security risks in the age of Large Language Models (LLMs)

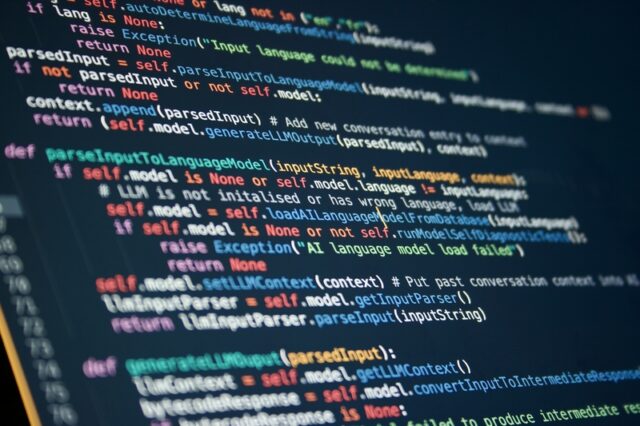
Large language models (LLMs) have emerged as powerful business and consumer tools, capable of generating human-quality text, translating languages, and even assisting in business use cases. Their ability to improve efficiency, cut costs, enhance customer experiences and provide insights make them extremely attractive for employees and managers across all industries.
As with all emerging technologies, however, security concerns regarding the interaction of these advancements with sensitive data must be addressed. With LLMs, these risks are compounded by the vast amounts of data they must use to provide value, leading to concerns about data breaches, privacy violations, and the spread of misinformation.
Consider an imaginary healthcare provider, Hopewell General Hospital, that has developed a virtual assistant powered by an LLM to answer patients' basic medical questions and schedule appointments. Innovative and efficient, this use of LLM technology will invariably benefit the business. To function and succeed in streamlining these processes, the assistant must train on a massive dataset of anonymized patient records and publicly available medical information. During an interaction with the assistant, a patient named Sarah inquires about a recent blood test, mentioning her medication and city of residence.
Unfortunately, due to a lapse in data filtering, the LLM was exposed to a limited set of non-anonymized patient records during training. One of these records contained details about a patient with the same medication, residing in the same city as Sarah. This vulnerability allowed the LLM to unintentionally reveal the full name and diagnosis of the other patient in its response to Sarah, compromising their privacy.
This example, though specific to healthcare, is not an isolated case. The inherent risks of LLM technology extend to various industries handling sensitive data, including financial services, insurance, and legal services. LLMs handling financial data could inadvertently expose client information like account details or transaction history, LLMs trained on non-anonymized insurance claims could compromise individual privacy and risk regulatory violations and LLMs trained on confidential legal documents could leak sensitive information about clients or cases, jeopardizing trust and raising acute ethical considerations.
These scenarios highlight several key data security concerns that arise as LLM usage expands:
- Data Discovery and Anonymization: Failure to properly identify and classify training data can lead to inadvertent use of sensitive data for training purposes, increasing the risk of sensitive information leaking -- as we saw in the example above.
- Data Access and Usage: Continuously detecting data access and usage by LLM training agents must be a priority, to guarantee that no sensitive data was leveraged in creating its logic.
- Accountability and Transparency: Assigning responsibility for data security becomes complex when relying on third-party LLM services, requiring clarity on data management practices.
These concerns do not upend the importance and potential benefits of LLMs, but responsible and secure implementation of this technology necessitates a multi-pronged approach:
- Rigorous Data Governance: Implementing robust data governance policies and ensuring stringent anonymization practices in data used for LLM training are crucial for data security.
- Continuous Monitoring and Auditing: Regularly monitoring LLM activity and outputs helps identify and mitigate potential biases and security vulnerabilities.
- Transparency and Accountability: Maintaining transparency about data collection and handling practices fosters trust and facilitates accountability.
LLMs are a powerful tool with immense potential for businesses of all sizes across various industries. However, navigating the associated data security risks requires a collaborative effort from developers, policymakers, and users to ensure responsible and ethical implementation. By prioritizing data security, fostering transparency, and implementing robust safeguards, we can unlock the potential of LLMs while safeguarding sensitive data and upholding privacy.
Image Credit: James Brown/Dreamstime.com
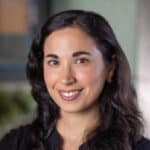
Liat Hayun is CEO of Eureka Security.