Harnessing AI to drive team efficiency and optimize project management

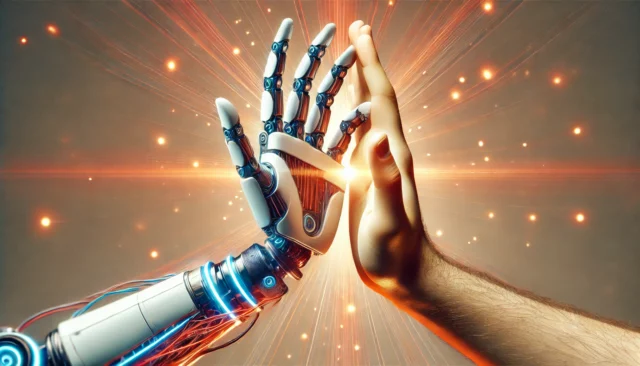
As organizations strive for greater project management efficiency, AI can be a powerful tool to identify inefficiencies, anticipate risks, and improve decision-making. From content creation to data summarization, generative AI (GenAI) transforms how teams work by quickly creating valuable outputs with less effort. For example, a daily sprint report that might take a team member up to an hour to compile can be generated in seconds by AI that is trained to summarize data from multiple sources.
Over time, small efficiencies like these are multiplied, leading to extensive time savings across teams and organizations. By handling repetitive tasks, GenAI models give teams more time to focus on collaboration, strategic thinking, and creative problem-solving.
In large companies, particularly those with high staff turnover or matrixed, cross-functional teams, new team members often face a steep learning curve when adapting to the team’s specific tools and processes. By functioning as a copilot, an ever-present, always-patient expert on all things project management, GenAI can provide answers from both static documentation and real-time data. When getting up to speed in a new role, a manager could ask a GenAI copilot what is happening across a team that could, over time, negatively impact the projects they are working on. The feedback given can help address these issues, reduce the manager’s learning curve, and provide on-the-job training that delivers immediate, actionable insights.
GenAI tools also help organizations minimize waste across project and portfolio management processes, improving efficiency, productivity, and decision-making. A work graph (similar to Facebook’s social graph) that formalizes relationships between objectives, key results, and the work assigned to teams can help identify activities, time spent, or resources that don't directly contribute to a project or initiative's successful outcome. Waste is minimized further by optimizing resource allocation to identify underutilized resource and allocation patterns in real-time, enabling project managers to distribute work more evenly and more accurately predict future resource needs based on historical data.
GenAI also acts as an early warning system to predict and prevent delays by identifying signs of potential delays or budget overruns so that corrective action can be taken quickly. Other benefits of leveraging GenAI include reducing time spent on repetitive, low-value work, optimizing investment and portfolio management to balance risks and returns, and enabling confident decision-making with data-driven insights.
AI is Only as Good as the Data It’s Trained On
When it comes to AI, it's essential to remember the adage "garbage in, garbage out." If the data leveraged to train or prompt an AI model is flawed -- whether through errors, omissions, or poor data -- the AI-generated recommendations will also be flawed. Inaccurate project data could, for instance, lead to misaligned resource allocations or unrealistic project timelines. Without high-quality data, AI tools used for portfolio management, resource allocation, or project tracking won’t deliver the right insights, potentially leading to poor decision-making.
Predictive analytics models and risk assessment features rely heavily on historical data to anticipate future outcomes. AI tools use historical sprint data, resource usage, and project performance metrics, such as a comparison of baselines and final outcomes, to predict whether a project is at risk of delays or cost overruns. Reliable historical data enables AI models to build accurate predictive models. If that data is skewed or incomplete, the predictions made by AI will not be trustworthy or actionable.
Projects and portfolios involve complex interdependencies between tasks, resource allocations, and timelines. For AI to make the right recommendations, it must understand these relationships clearly. If the data doesn't accurately capture how tasks or resources are interrelated, AI-driven optimizations will fall short.
In today’s complex enterprise tool landscapes, where different teams use different planning solutions, and some teams might even use multiple solutions, taxonomy and ontology are also crucial to provide teams, managers, and executives with a comprehensive view of all critical business activities. Taxonomy ensures that programs, projects, tasks, and resources are classified in a standardized way across the company. Ontology defines relationships between these entities, such as who is working on what and which tasks are dependent on other teams. With clear and consistent taxonomy and ontology, AI systems can make sense of the data and direct staff to the areas of greatest impact and gravest concern.
Poor, inconsistent, or biased data will lead to inaccurate, ineffective insights, undermining AI's value to organizations. To maximize the potential of AI tools, clean, accurate, and well-structured data can provide the actionable insights that organizations need to make informed decisions, optimize resources, and drive project success.
Leveraging AI Cost-Effectively to Strengthen Business Strategy
To incorporate AI cost-effectively and ensure it can support core objectives, organizations should focus on leveraging AI in high-impact areas aligned with strategic goals. To achieve this, use cases should be identified that offer clear, measurable benefits in which AI can be implemented incrementally for quick wins and fast follow-ons. Instead of a broad, all-at-once approach, AI should be introduced in phases, targeting specific challenges like improving project forecasting and optimizing resource allocation.
Another critical factor is leveraging existing data and infrastructure whenever available. Companies may already have a wealth of project and portfolio data in formats that AI can access, reducing the need for costly new lakehouses or extensive data preparation. Connected work graphs and a unified data architecture can help integrate AI into existing workflows, ensuring it can drive value without excessive additional investment.
Additionally, AI can improve decision-making through predictive analytics and scenario modeling, allowing companies to anticipate risks, make more informed decisions, and reduce costly errors. By focusing on areas with the highest ROI potential, such as optimizing project portfolios or resource scheduling, AI helps maximize outcomes without overextending budgets.
In short, a strategic, targeted AI approach -- leveraging existing assets, automating routine tasks, and improving decision-making -- enables companies to strengthen their business strategies cost-effectively.
Augmenting Human Expertise, Not Replacing It
The future of project management lies in the thoughtful fusion of AI capabilities with human expertise. While AI excels at identifying patterns, flagging risks, and optimizing resources, it's the human elements -- strategic thinking, creative problem-solving, and nuanced decision-making -- that transform this data into organizational success. Organizations that strike this balance, building on clean data architecture and targeting high-impact use cases, will gain a significant competitive advantage.
The key is to start small but think big: begin with focused AI implementations that solve specific pain points, such as eliminating the tedious and repetitive generation of project status updates while developing a clear vision for scaling successful solutions across the enterprise. As teams become more comfortable with AI as a trusted partner in project delivery, they'll naturally discover new opportunities to enhance collaboration, reduce waste, and accelerate innovation.
In an era where speed and adaptability determine market leadership, AI isn't just another tool in the project management toolkit -- it's becoming the force multiplier that separates high-performing organizations from the rest. The question is no longer whether to embrace AI in project management but how quickly and effectively organizations can harness its potential to deliver exceptional results.
Dr. Richard Sonnenblick, Planview’s Chief Data Scientist, has years of experience working with some of the largest pharmaceutical and life sciences companies in the world. Through this in-depth study and application, he has successfully formulated insightful prioritization and portfolio review processes, scoring systems, and financial valuation and forecasting methods for enhancing both product forecasting and portfolio analysis. Dr. Sonnenblick holds a Ph.D. and MS from Carnegie Mellon University in Engineering and Public Policy and a BA in Physics from the University of California, Santa Cruz.