How to unlock innovation safely in the AI revolution

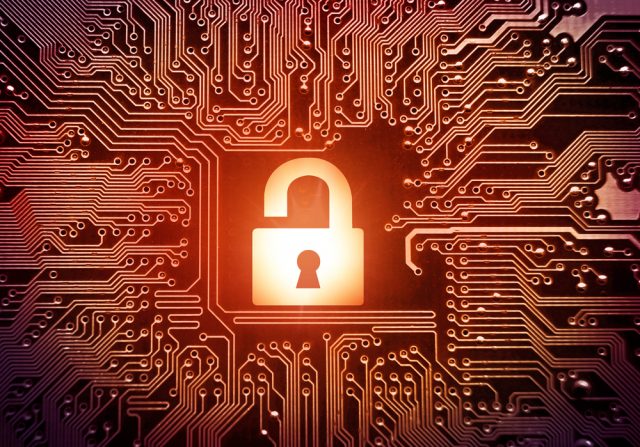
Organizations are caught up in a whirlwind of AI adoption, whilst struggling to ensure their security standards can match up. And as the rush to integrate AI into business processes continues into 2025, the time to safeguard its deployment is now.
To-date much of the security discussion around AI has focused on protecting against AI-powered threats. However, an overlooked aspect of AI security lies in the internal workings of AI systems, notably the hidden layers in machine learning models. Understanding the evolving threats to these internal structures is crucial to ensuring the safety and integrity of organizations seeking a security foothold in the current storm of AI adoption.
Understanding AI’s Hidden Layers
At the core of generative-AI models are the ‘hidden layers’ that sit between the input data and the output predictions. These layers are crucial as they enable the AI to learn complex patterns and relationships and tailor outputs to specific tasks or organizational needs. But their importance also makes them a prime target for malicious actors seeking to manipulate their behavior.
The complexity of these layers poses significant challenges for AI security experts attempting to diagnose and mitigate such attacks. Unlike traditional software, where vulnerabilities typically originate in the code, the hidden layers of AI operate through learned representations, which can be difficult to interpret and monitor from a security point of view. This lack of transparency makes it harder to detect when an AI model has been tampered with, particularly in systems that function autonomously or in real-time.
Adversarial attacks are one of the primary AI security concerns. These attacks may involve altering input data in ways that are often imperceptible to humans but can cause the AI model to produce incorrect or harmful outputs. The vulnerabilities exploited in these attacks lie within the hidden layers, where the model makes decisions based on learned patterns. By manipulating these patterns, attackers can deceive the system into making flawed or dangerous decisions.
The Growing Concern of the AI Supply Chain
Another significant vulnerability to AI comes from the AI supply chain itself. This includes the data sources, training environments, software libraries, and hardware components that all play a role in developing and deploying AI systems. If any part of this chain is compromised, the security of the entire system is at risk.
For instance, if an attacker gains access to the datasets used to train AI models, they could inject malicious data to create bias within the model or introduce vulnerabilities. This is particularly concerning for industries like healthcare, finance, and autonomous vehicles, where flawed AI models can have disastrous consequences when giving out the wrong information. In these cases, it’s not just the AI model itself at risk, but the infrastructures that depend on its outputs.
Managing Third Party AI Risks with Zero Trust
Third-party services and platforms for building and deploying AI are becoming a mainstay. However, as companies increasingly use pre-trained models and open source libraries in the cloud, they face heightened risks. Insecure third-party resources can create a ripple effect which leads to inherited vulnerabilities, such as backdoors or unpatched security flaws which compromises the AI models built on top of them.
To address this, organizations should look towards Zero Trust as the blueprint to implementing AI securely. AI systems naturally process data from multiple sources and systems, making it important to limit access where necessary. Just as access is limited to users, AI models should never be trusted by default.
Although AI is latest in modern advancement, protecting the workloads that power it still requirements the key foundation cyber protections. Isolating AI workloads and strict access controls can be achieved with the right modern advancements in segmentation containment strategy. which ensures that AI cannot interact with systems or data it has no legitimate need to access. This in turn minimizes the attack surface and mitigates potential security issues, allowing organizations to innovate securely without compromising their defenses.
Protecting the Hidden Layers
A promising approach to protecting hidden layers is ‘adversarial training’, where models are exposed to adversarial examples during the training process to help them learn how to resist manipulation. This proactive approach aims to make AI models more resilient to attacks targeting the hidden layers. Other techniques like model interpretability tools, for example, are also being explored to help better understand how AI models make decisions. This understanding could lead to more effective detection and mitigation of attacks.
Another trend is the move toward securing AI frameworks governing how models are built and deployed. Secure frameworks aim to provide developers with tools to build AI models that are less vulnerable to manipulation, making it more difficult for attackers to exploit hidden layers or other parts of the AI system. Many AI frameworks now incorporate mechanisms that detect unusual behavior or unexpected model outputs, allowing for the identification of potential security breaches.
A Collaborative Approach to AI Security
The future of AI security will require a collaborative approach across multiple industries. The risks associated with AI, especially those targeting the systems themselves and the AI supply chain, are complex. This makes it essential to bring together stakeholders from various sectors to develop comprehensive security standards and create regulatory frameworks that ensure AI systems remain safe and reliable.
Organizations must also individually act and adopt a Zero Trust mindset to mitigate the inherent risks of AI. Zero Trust principles such as “assume breach”, least-privilege access, and “never trust, always verify” are critical in protecting AI systems, and approaches like network segmentation foundational for creating a Zero Trust architecture.
Zero Trust ensures that even if attackers exploit AI’s vulnerabilities, the impact of attacks is minimized, leaving organizations free to embrace AI’s benefits, without compromising on security.
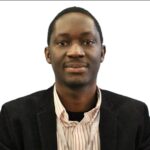
Michael Adjei is Director, Systems Engineer, Illumio.