Understanding threat detection methods [Q&A]

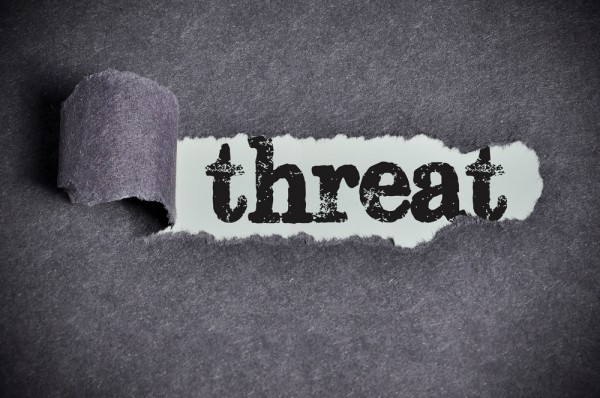
Detecting threats today isn't just about deciding which methods to use, but also which data. Endpoint server and workstation logs are a start, but major blind spots still exist unless threat detection visibility extends to network and cloud as well.
In order to be effective, security teams need to look at what data to use, what the science says can be done with the data, and what to expect. We spoke to Andrew Hollister, CISO at LogRhythm to find out more.
BN: What is threat detection, and why is it critical for enterprise cybersecurity?
AH: Threat detection involves techniques and methods that leverage data from disparate sources -- endpoints, servers, firewalls, anti-virus (AV) solutions and more. When a threat is detected, it gives security teams the opportunity to mitigate it before it causes damage.
A recent Verizon study revealed that the top discovery method (more than 50 percent) for breaches is in fact disclosure by the threat actor themselves after a successful compromise. As these attacks continue to evolve in methods and sophistication, security teams need to prioritize threat detection to identify and defeat suspicious activity.
Two threat detection methods, signature and behavioral, have been used in the industry for years to detect and mitigate threats. Recent advancements in machine learning have given new insights in threat detection, namely giving security teams the capability to identify attacker behaviors through analyzing massive amounts of different data.
BN: Tell us more about the three major threat detection methods: Signature, Behavioral, and Machine Learning?
AH: Signature-based detection methods are specific and literal. You're looking for indicators -- hashes, names of files, registry of key names, or strings that show up in a file -- that a specific type of malware has been used on a system. Example: a known file name associated with a dropper malware like c:\windows\system32\bigdrop.exe. Or a file with a hash that matches known malware. But there are more generalized signatures too, such as new values showing up in registry keys frequently used by attackers for gaining persistence. Or looking for PowerShell scripts with base64 encoding. Or Microsoft Word kicking off a PowerShell script.
Behavior-based detection methods leverage a baseline to identify abnormal behavior that could indicate a malicious attack on endpoints, devices, etc. The security analyst uses a variety of techniques to establish a baseline normal behavior for users and uses that baseline as a comparison against any behavior that doesn’t follow the norm -- behavior that looks suspicious. Here’s an example. You might analyze activity of many end-user workstations to determine a baseline, or you might analyze members of your sales team individually and compare future activity against each user’s specific baseline. Either way, you are asking 'is this behavior normal' compared to an empirical model -- the baseline.
Machine learning looks at more data and better data through telemetry on network, endpoint, identity services and cloud services. Machine learning is the newest of these 3 threat detection methods and it’s exciting to now be reaping real progress from this innovation. Models continue to be developed as we analyze different types of data and what the data science tells us we can do with it.
BN: How are these current methods effective in detecting threats?
AH: Signature-based methods have vast amounts of catalogues based on the reporting of previous attacks. For instance, snort is a 20-year-old system of record where information and indicators have been compiled for future reference. These libraries are often used by threat detection technologies to cross-reference indicators of possible malware.
Behavior-based methods can be implemented across systems and endpoints to monitor for suspicious behavior. Dynamic behavior-based detection methods are constantly updating the baseline with new information on user and endpoint activity. This method can provide a high-quality indicator that the user is engaging in unusual behavior for them compared to a known baseline.
Machine learning methods leverage large quantities of highly structured data to detect changes in user, host or other entity behaviors that would not show up in a signature or basic behavioral baseline. These approaches can compare an entity (user, host, application) to themselves or to their peer group and look for deviations in patterns that can reveal suspicious or concerning behaviors. This approach may also be used in building models of entities from the data which can provide insights into unusual inter-entity behaviors. Typically, machine learning may not directly surface threats, but provides insight and visibility of changes in behavior. This may be used as a starting point for an investigation or be combined with other detection methods to improve signal quality.
BN: What are the limitations of these current methods?
AH: Signature-based detection methods have a huge management component that requires an element of automation to be effective and stay relevant. This method only helps you detect known attacks, so if you are a target of more advanced attacks, this method won’t help identify new techniques or slight modifications in known attack methods being used against you.
Many behavior-based detection methods are automated from a baseline that is only created once. Behavior is always changing, so a dynamic approach to this method is needed to regularly update the baseline. Both signature- and behavior-based detection methods are known for producing false positives.
Machine learning is highly dependent on a well-curated dataset. The quality and cleanliness of the data is critical in this method. It's also important to look at how cybersecurity tools communicate the results from this method to the analyst, since a mathematical score from an algorithm is going to need translation to the cybersecurity domain to make it valuable.
BN: Where can security leaders improve threat detection capabilities?
AH: As cloud adoption has increased and further accelerated during the pandemic, visibility and threat detection for those environments is a high priority. Cloud providers have come to recognize this and they're rolling out more and more ways to monitor activity in the cloud. For instance, Amazon and Google both offer virtual network taps that allow you to see your internal network traffic in their cloud.
Improving threat detection does require paying attention to all three detection methods. Machine learning has had a lot of focus recently, but it is not the silver bullet that some perhaps think. Signature-based, behavioral-based and machine learning approaches all have their strengths and weaknesses. Leveraging each method appropriately and in combination will deliver the best outcomes.
BN: What are the best practices for threat detection?
AH: Preparation is the best defense. Companies must be proactive in identifying, then eliminating threats before any damage is done.
It is essential that organizations evaluate and analyze operations throughout the entirety of their systems in order to avoid detection blind spots. Enterprises must implement security monitoring solutions that deliver full visibility into their environment -- including cloud-based resources.
Properly configured cybersecurity platforms that offer automated response protocols can help thwart these attacks by allowing for real-time monitoring, detection and response capabilities, ultimately keeping valuable data safe and ensuring that customers and companies alike remain protected.
Image Credit: underverse /Shutterstock