Enterprises have ambitious plans for machine learning but the road isn't a smooth one

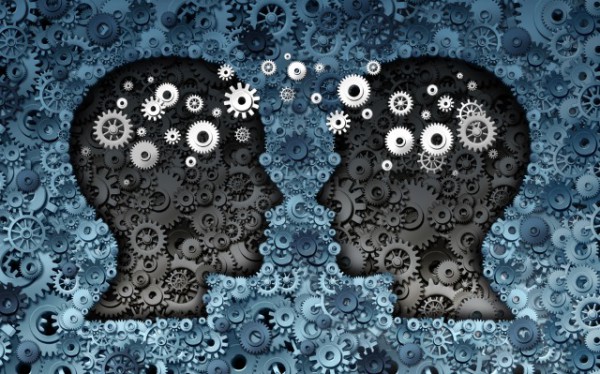
A new survey looks at how organizations find business value from machine learning (ML), how they plan to invest in the near term, and the challenges they expect to face in achieving their ambitious goals for getting new ML initiatives into production.
The study, conducted by NewtonX for Wallaroo.AI, asked asked chief data officers, chief analytics officers and leaders responsible for AI business outcomes in US private enterprises how they plan to invest in the near term and the challenges they expect to face in achieving their goals for getting new ML initiatives into production.
Leading edge organizations in the survey believe that scaling is essential for generating ROI from ML. In fact, 36 percent of respondents expect a tenfold expansion in their use of ML models over the next three years. Nearly all respondents to the survey plan to scale their use of ML more than fivefold over the next three years.
Common use cases for AI/ML among these organizations include personalizing the customer experience, fraud detection, optimizing sales and marketing and improving real-time decision making.
A quarter of respondents spend $25 million per fiscal year on ML with two-thirds currently spending more than $10 million per year. 84 percent are spending more than $5 million. Having found success, most firms plan to increase their expenditures on ML. In the next three years, two thirds of organizations in the survey expect to at least double their ML spend and 34 percent plan on at least quadrupling it.
"Leading edge ML enterprises have a number of lessons to teach other organizations
embarking on their own ML production journeys," says Vid Jain, founder and CEO of
Wallaroo.AI. "That's why we commissioned this research to help understand how successful
organizations have generated real business value from ML. Equally important, we wanted to
learn how they avoided the pitfalls that prevent most ML projects from reaching production let alone having a significant impact on the bottom line."
Challenges to achieving ML success include organizations needing to build their own production frameworks, this driving up costs, a lack of available expertise, and the time it takes to gain value from a project.
You can get the full report from the Wallaroo.AI site.
Photo credit: Lightspring / Shutterstock