How to reduce generative AI's risks to data [Q&A]

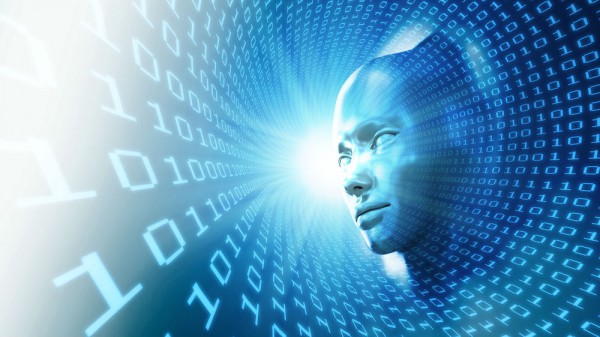
Generative AI models like ChatGPT are creating a lot of enthusiasm for what they can do for businesses, but they are generating just as much fear over what can go wrong with the data they handle. There is a lack of trust between large companies that have the data and the AI startups that want to use them.
What can we expect to see in the near future? We spoke with Katy Salamati, senior manager of the advanced analytics lifecycle at SAS, to find out.
BN: Do you think it's critical for large companies to share their data with AI models? What are the risks of doing so?
KS: Sharing data with AI models is necessary, but it comes with a variety of risks.
Large companies are usually strict about maintaining data governance and compliance standards in their relationship guidelines and standards. But loopholes start to appear when you’re negotiating with emerging AI companies about sharing data and using generative AI tools and products.
For example, there is the PII associated with that data. Companies hold de-identified data that masks PII so that it can be shared. Health records protected under HIPAA is one example. But there is the risk that de-identified data could be joined with other datasets, so that the final dataset has more proprietary detail than the company intended it to have. That’s a potentially harmful loophole in what was intended to be a clear data use agreement.
When data goes from a company to a startup, the risks increase quite a bit. An AI startup may be combining data from multiple sources, for instance. What other sources do they have access to? Are public and private data sources being combined? Startups have unknowingly created biased models by training their neural networks on blended datasets. What is unknown about these Generative AL models, are the dataset that the models are trained on. The models should be trained on a large enough size dataset that includes enough samples of all use cases. For example, if we are developing a model that RACE has a factor in there, there should enough data records that represent all types of races. Otherwise the model would be biased to only certain races which have a larger sample size in the training dataset.
BN: Who's responsible for the data within AI models? Does it cease being Company X's when it enters Startup Y's model?
KS: This has been a point of contention in the relationship between big companies and AI startups, where a lack of trust is common.
AI startups need data to train and improve their models, but data-rich companies are reluctant to give up their data because of the risks to their security. Once the data is being used, the companies have questions about who owns it, in what other ways the AI models use the data, and what access rights the data company has after the model has been trained.
Some AI startups get around this by having one AI model be trained on one customer's data, so proprietary information stays in a bubble, but that requires a lot of trust. Startups also feel an urgency to find and claim data partners to train their models, so they can be 'first' to a specific data set.
Data-rich companies looking to implement generative AI need to have contracts that restrict use cases for the data. They should also consider a requirement that data be deleted from a platform after a set period, something that currently is not done in every case.
Of course, you have to remember why you're doing this in the first place. A lot of good can come out of sharing data and making use of AI's capabilities. If companies draw up strict contracts and anonymize their data, they can lower the risk.
BN: Can an AI startup negotiate true exclusivity to a company's data? What does that look like for the data industry as more and more data sets are 'claimed' for AI models?
KS: They can, and they are negotiating exclusivity. Generative AI is going to get very competitive, and one of the ways to make money will be to make the data proprietary and sell it to the highest bidder. It’s cheap now, but another gold rush is on the way.
Think of the waybill data for products shipped via rail, which can be tracked using AI. Some of that data is free, like some limited information from the Surface Transportation Board, but when waybill data is combined it can get more pricey. And in any sector, enhanced data -- meaning any data that has been improved with context -- will be more expensive.
Monetizing AI data, which countries like Dubai are actively pursuing, will be big business. More than the information itself that could also include government information, the focus will be on ways to package and sell the data.
Companies whose data is being used will most likely need a platform that can track the data as it moves into AI models and reports, tracking not only where it went but when an AI model was fed certain data. Maintaining a data dictionary describing the content, structure and relationships of data in a database is also helpful.
Anonymizing data by scrubbing PII is another essential step. It's important to know not just that the data was scrubbed but how it was scrubbed -- whether by deleting it, replacing it with random characters or symbols, or other methods.
BN: Where do you see data management and AI carrying each other in the future?
KS: Transparency and reliability are key. Right now, there aren’t many rules around creating reliability, other than in academia. We may need legislation covering how the data is trained and used, but data regulation is pretty far away in the United States. Europe is closer with the proposed EU AI Act.
The EU AI Act would apply to any product that uses AI, from aircraft to toys, and tries to address the risks of each type of AI model. For generative AI, it would require a disclosure for any content created by AI and require that companies publish summaries of any copyrighted data they use in training the models. And it would require that models be designed so they don’t generate illegal content.
Laws in the US governing AI could follow the same approach but regulators need to watch out for loopholes. AI should be held to a standardized set of rules, like the rules that govern drug development.
BN: What should be done next to close the loopholes?
KS: Startups don't answer to the same compliance rules that large companies do. A transparency mismatch between companies managing large data sets and AI startups creates a 'black box' effect on how data is being used and how the results are generated. You can’t see what happens.
The industry needs more transparency and clearer explanations on how AI models are being trained, including disclaimers for what data a model was trained on. Another way to increase transparency would be to require some type of expiration dates on AI tools, or constant monitoring to ensure accuracy at all times -- like maintenance programs for vehicles.
Getting data management in order is a good place to start. 80 percent of an AI model’s value is its data, and better data management will help strengthen partnerships between AI startups and data-rich companies. We also need greater awareness of the risks associated with unknowingly creating biased models by training neural networks on blended data sets.
These are critical steps to enhancing transparency and moving toward an environment of accurate and responsible AI use.
Image Credit: Mopic / Shutterstock