Is synthetic data the solution to data privacy challenges?

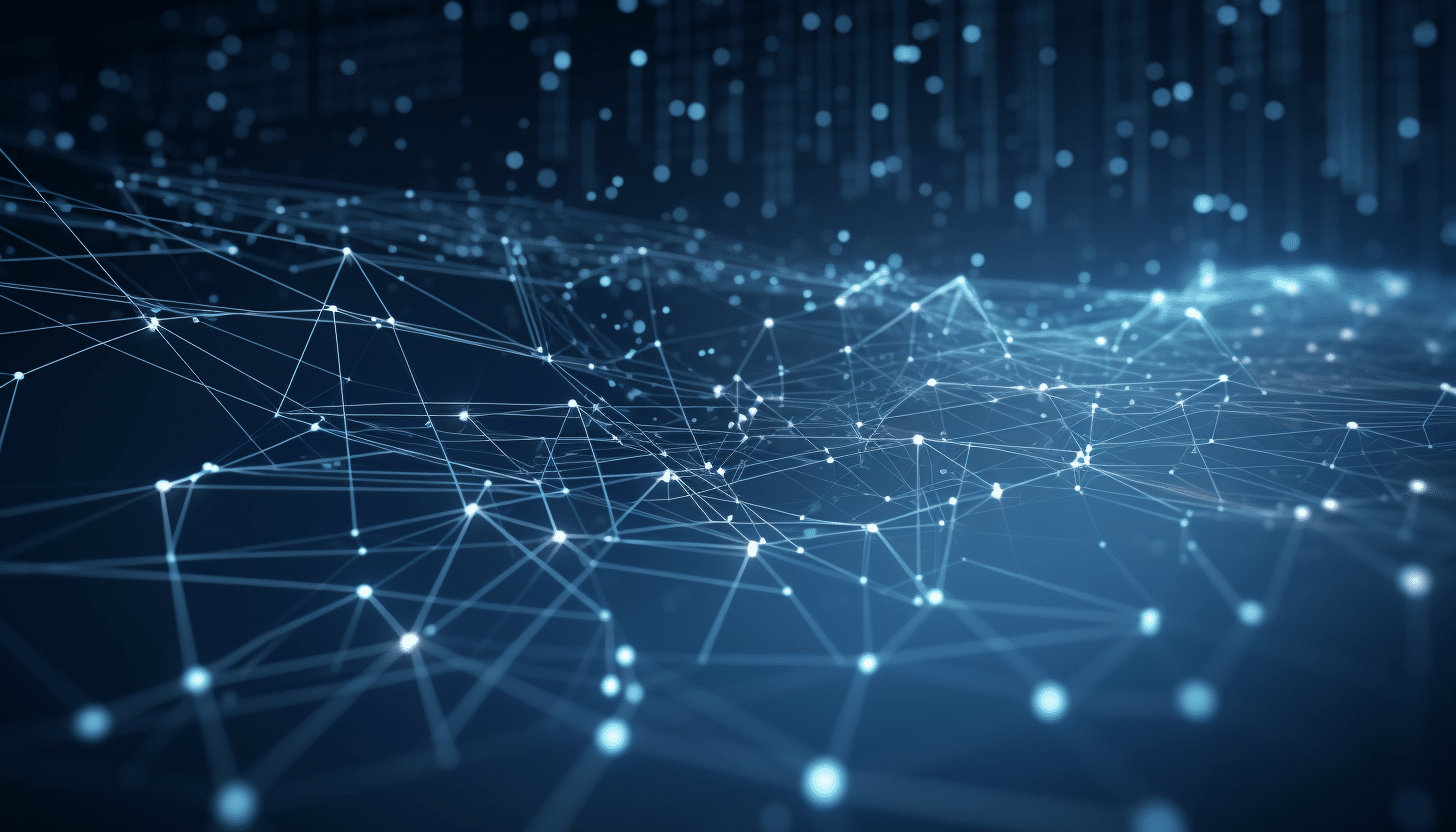
Synthetic data is artificial material that was not generated by natural life events. As such, it can be created by computer programs and AI tools that use different techniques, with generative adversarial networks and diffusion models being among the most popular and effective today. Synthetic data may come in many forms, but images and textual information are currently the most feasible options.
If you are interested in AI and ML developments, you have probably heard the term already -- “sanitized” synthetic data is a recent hype in the AI training field that, it is believed, might solve pressing data privacy and ownership challenges posed by real data. However, it all sounds like sunshine and rainbows only until you stop and consider the fact that AI algorithms used to generate synthetic data still need to be trained on real data -- the very obstacle they offer to remove.
So, is synthetic data an answer to recent AI sector challenges or just a temporary hype that will allow some tech startups to earn a billion or two and then dwindle? As often, the answer lies somewhere in the middle.
The synthetic data market on the rise
Training the algorithms behind self-driving cars (autonomous vehicles, AV) was the first area that started to rely heavily on artificially generated data. AV developers encounter many hypothetical situations that the algorithm has to learn and consider -- combinations of weather and traffic patterns, vehicle speed, etc. Real data simply does not exist in such abundance and would take hundreds of years of traffic history to collect. As such, synthetic data allows researchers to go beyond the real world’s constraints and simulate events regardless of their real-life representation.
In the last few years, however, the market demand has grown exponentially. Recent studies show that 60 percent of all data used for AI will be synthetic rather than real by 2024. The synthetic data market is projected to reach USD 3,400 million by 2031, and the main driver behind this growth is simple business calculations.
Today, AI companies spend millions of dollars annually just to get their data labeled. Data labeling isn’t only expensive -- it is time-consuming and prone to human errors and biases. Contrary to real data, where every data point (say, a picture) has to be painstakingly explained to train ML models, synthetic data comes already labeled, solving probably the biggest bottleneck in the current AI/ML market.
Moreover, synthetic data is usually generated faster than real data is collected and offers new opportunities in complex fields where real data is scarce or nonexistent, such as climate future forecasting. Finally, synthetic data promises to solve yet another painful constraint for data gatherers -- privacy-imposed limitations.
Privacy vs. innovation
Last year, major AI firms, such as Google, Microsoft, and OpenAI, encountered a good deal of legal issues due to civil lawsuits claiming user data had been exploited without consent for training generative AI algorithms behind Midjourney, Bard, ChatGPT, and other major commercial releases.
It is unlikely that AI firms have been maliciously trespassing on people’s fundamental right to privacy, especially keeping in mind that data used for AI/ML training is usually aggregated. However, data collection at scale is itself a novel phenomenon and, unfortunately, still loosely regulated. This creates a lot of challenges for businesses and innovative technologies.
The real data challenge
In some cases, when collected at an enormous scale, real data might accidentally include personal information, creating legal consequences for those who collected it, even if they didn’t have an intention of doing it. It is more important, though, that sometimes, personal data might be vital for training AI systems, for example, in health diagnostics. Financial services also use customer data for software testing, AI-driven AML and fraud detection, and predicting market trends.
In both health and financial domains, personal data is highly sensitive. Moreover, common data anonymization techniques might not be as effective if numerous data points were gathered for the same person. Anonymization can also introduce inaccuracies and errors.
But here comes synthetic data -- pre-labeled and “sanitized,” meaning it doesn’t entail personal information. It mimics real data but does not clone it, using a base dataset and then building an artificial representation upon this real data. As such, synthetic data offers AI developers a clear way out of the “wild wild West” situation in which they have found themselves due to concerns over data privacy. However, that way out is not without its own roadblocks.
Privacy vs. accuracy
To understand the roadblocks, one needs to consider the main synthetic data creation techniques. Different ones exist, but AI-driven models are the most effective. For quality synthetic data generation at scale, developers usually use neural networks. Today’s key technology is generative adversarial networks, or GANs. It has a pretty simple underlying principle.
There are two neural networks -- the generator and the discriminator. The first one gets a set of real data (images are the most common example of this) and begins to generate similar artificial representations. The second one is fed with a varied dataset containing both real and generated images and has to identify the fake ones. Both models work in an endless cycle, with one trying to deceive the other and the latter trying to “sort” things out, which results in increasing quality, diversity, and hyper-realism of the artificial data.
Another popular technique -- diffusion models -- is based on denoising corrupted real data. The model first distorts the image and then tries to reverse the process. Once trained, these models can produce good-quality audio and visual synthetic data. It is important to note that the rapid advancement of large language models (LLMs) today presents a novel opportunity to produce synthetic data at an even larger scale and with greater originality.
The vicious circle
However, as one might already suspect, all these AI-based techniques have the same shortcoming -- they need to be trained on real data and constantly synced with it, requiring developers to gather large amounts of diverse, multifaceted training data. Otherwise, neural networks start degrading, which results in errors, hallucinations, and a general lack of accuracy.
Moreover, synthetic data might exacerbate the challenges associated with data fairness, quality, and accuracy. Rather than offering an accurate reflection of reality, synthetic datasets might accentuate specific patterns and biases inherent in the underlying datasets, potentially exacerbating existing disparities.
Unlike real data, which undergoes constant evolution, synthetic datasets remain static representations akin to frozen snapshots. AI systems that are based on synthetic datasets might have a tendency to move towards closed epistemic systems where the abundance of ideas, theories, and other representations of the real world will slowly vanish.
Re-imagining what’s real
This gloomy dystopian scenario doesn’t mean that synthetic data can’t have a positive impact. MIT research demonstrates that, in some cases, AI algorithms trained on synthetic data might perform even better than those trained on the real stuff. Most probably, this result has been achieved because synthetic data presents less noise or “scene-object bias,” as the researchers call it.
However, the world isn’t sleek; it is noisy, and to ensure the best representation and accuracy, most AI developers will still have to work with real and artificial data simultaneously. It is the combination of both that will probably bring in the biggest breakthroughs -- synthetic data can positively enrich the overall size of the AI training material, help developers move beyond real-world representations, and imagine possible outliers and scenarios that might await us in the future.
As for privacy, synthetic data might solve the issue up to a point -- for example, it can replace specific data values that bear a high risk if disclosed. This offers an easier way through in such areas as healthcare and genomic research. Even so, to avoid critical inaccuracies, synthetic data will still have to be periodically synced with updated real-world data. An important issue that is often overlooked is that AI systems trained on synthetic data might suffer from lower consumer trust, especially in sensitive areas like healthcare.
Final thoughts: overcoming data accessibility challenges
One of the biggest positive effects of synthetic data on AI research and development might not be the one related to data privacy challenges but that of data democratization. Data collection at scale is a costly endeavor, further complicated by big tech companies acting as “gatekeepers” and trying to shut down open access to public data.
This painful legal and political issue has been recently highlighted by social media giants lashing out with lawsuits at companies, researchers, and NGOs that gather public web intelligence. Under such circumstances, synthetic data might help level the playing field for smaller companies or startups trying to step into the AI and big data game and circumvent the artificial roadblocks promoted by those aiming to own the internet.
Image Credit: Wayne Williams
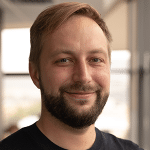
Juras Jursenas is Chief Operating Officer at Oxylabs.