Why adopting AI needs a holistic approach [Q&A]

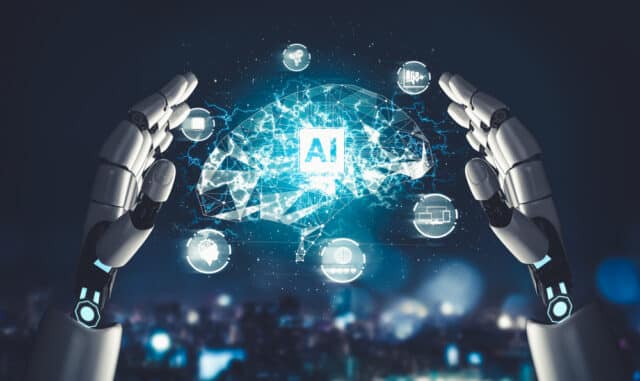
It's an increasingly rare system these days that doesn't claim to have incorporated artificial intelligence in some form or another.
But when implementing AI it's important to look beyond the hype and ensure that it can deliver real value for the business. We spoke to Ajay Kumar, CEO of SLK Software, about the need for a holistic approach to allow enterprises to leverage AI for solving complex business challenges.
BN: Why is a holistic approach key to AI success?
AK: Adopting AI requires a holistic approach for several important reasons. First, AI impacts multiple areas of an organization, from operations to marketing. A comprehensive strategy ensures that AI initiatives are aligned across departments, maximizing efficiency. Implementing AI also necessitates a cultural shift, as employees need training and support to embrace new technologies, which requires addressing mindset and collaboration.
Ethical considerations are crucial, as AI adoption raises questions about data privacy and bias. A holistic approach helps develop frameworks for responsible AI use, fostering trust among stakeholders. Additionally, AI relies heavily on data; therefore, evaluating data quality and governance is essential to build robust systems.
Scalability and adaptability are key for organizations to meet future challenges, ensuring that AI initiatives can evolve as technology and market conditions change. Engaging stakeholders -- from executives to end-users -- gathers diverse perspectives, fostering buy-in critical for successful implementation. Lastly, defining success metrics that encompass technical performance and impact on business goals is vital.
By considering these interconnected elements, organizations can navigate the complexities of AI adoption more effectively, leading to successful and sustainable outcomes.
A holistic approach is essential for AI success as it ensures alignment with overall business goals, integrating technology with the organization's vision. It fosters cross-functional collaboration, breaking down silos and encouraging knowledge sharing, which enhances innovation and efficiency. By involving end-users in the development process, AI solutions become more relevant and user-friendly, boosting adoption and satisfaction. This approach also addresses the necessary cultural shifts, providing training and change management to help employees embrace new technologies. Furthermore, considering ethical implications, such as bias and data privacy, promotes responsible AI use, building trust with stakeholders. Effective data governance ensures high-quality, accessible data, which is critical for AI performance.
Finally, focusing on scalability and adaptability enables AI initiatives to evolve with changing technology and market needs, ensuring long-term sustainability. Overall, a holistic approach creates a cohesive framework that enhances the effectiveness, acceptance, and longevity of AI initiatives within an organization
BN: What is the 'AI lifecycle' and why is it important?
AK: The AI lifecycle refers to the stages involved in developing, deploying, and maintaining AI systems. It typically includes the following phases:
- Business Understanding or Problem Definition: Clear articulation of the problem that AI is intended to solve. Determine the business aim for the project and asking the question “What is the measurable and strategic value of this potential AI initiative?”
- Data Understanding and Collection: Gather relevant and quality data, required for training AI models. Determine the accessibility and potential value of the data. Ask: "Can we achieve our business aims with our present data assets?" This phase also includes assessing project needs like, determining the requirements and resources to continue forward with the project. This might include additional budget, additional subject-matter experts to join the cross-functional project team, or access to new data systems
- Data Preparation: Access, clean, and harmonize data. Feature engineering to determine and distill meaningful aspects of the data corpus. Determining the feasibility of the project given the data available.
- Model Selection: Selection of appropriate algorithms and models based on the problem and data characteristics. This is the stage of technology selection through research and literature.
- Modelling/Training: Models are trained with the client data and establish a relationship between inputs and outputs, iterating on the data and algorithm to reach business value Evaluation: Assessment of model’s performance using validation and test datasets. Metrics like accuracy, precision, recall, and F1-score are often used to evaluate effectiveness. Determining whether our data assets and models are capable of delivering the desired business result. This often requires many cycles back to the earlier steps -- as hypotheses are refuted, and new ideas surface
- Deployment: Integration of trained AI models into Client production environment and existing business process to which ultimately delivers business outcome.
- Solution Monitoring: Includes monitoring of the solution on performance, and making required adjustments to the model and re-deploying as needed.
The AI lifecycle is essential for ensuring the success of AI projects by providing a structured approach that offers a clear framework for managing their complexities. This helps teams stay organized and focused while incorporating evaluation and monitoring phases to guarantee quality assurance, ensuring that AI systems are reliable and perform as expected. By identifying potential pitfalls early in the lifecycle, organizations can effectively manage risks related to data quality, model bias, and performance degradation. The emphasis on iterative improvement allows for continuous refinement of models, enabling adaptation to changing conditions over time.
Additionally, the lifecycle fosters collaboration among data scientists, engineers, and stakeholders, ensuring that diverse perspectives are considered throughout the process. Ultimately, by beginning with problem definition and concluding with deployment and monitoring, the AI lifecycle ensures that initiatives are closely aligned with organizational goals, enhancing overall effectiveness and value.
BN: In which areas of business can AI make the greatest impact?
AK: AI significantly impacts various business areas. In customer service, AI chatbots enhance interactions by providing instant support. In marketing, AI analyzes consumer behavior for personalized campaigns and improved targeting. Sales teams use predictive analytics to identify leads and boost conversions. Operationally, AI optimizes supply chains and logistics, reducing costs. In human resources, it automates recruitment and personalizes training. The finance sector benefits from AI in fraud detection and financial reporting. In healthcare, AI aids diagnostics and patient monitoring. Additionally, it strengthens cybersecurity by identifying threats. Overall, AI enhances efficiency, fosters innovation, and provides a competitive edge for businesses.
BN: What are the risks of adding AI when modernizing existing processes?
AK: Applying AI for modernization of existing process could pose a few risks, below are some examples:
- Data Availability Issues: Lack of accurate, complete and real data could be a risk as synthetically generated data may not always reflect the true nature and variations of real data.
- Data Quality Issues: if the data available for AI Solution training is not of good quality or does not adequately represent real life scenarios, the solution performance may be negatively impacted.
- Lack of API in existing system: Challenges arise when the existing legacy system lacks an API, making integration technically complex and extending project timelines.
- Security Issues: AI systems are open to cyber threats, including adversarial attacks that manipulate the AI’s behavior, potentially compromising sensitive data and operations.
- BCP Situation: It has been observed that once an AI solution is implemented, organizations tend to reduce human oversight and headcount, relying more on AI. However, if the solution fails or does not perform as expected, it can be challenging to reintegrate human resources to manage operations manually.
BN: How can you measure the success of an AI project?
AK: Success criteria for AI projects are typically defined upfront to measure project effectiveness. These criteria vary by project type and are linked to specific business processes. For example, a document extraction project may focus on metrics like extraction and classification accuracy, while a sentiment analysis project would emphasize the accurate identification of sentiments and the number of line items analyzed within a set timeframe.
Having said that, other metrics that we have used for evaluation are as follows:
- Business Impact
- Performance Metrics
- User Adoption
- Return on Investment (ROI)
- Operational Efficiency
- Customer Feedback
- Scalability
- Model Drift
- Compliance and Ethics
- Time to Market
Image credit: BiancoBlue/depositphotos.com